Pallav Kumar SHRESTHA
PhD Researcher
Department Computational Hydrosystems (CHS)
Helmholtz Centre for Environmental Research - UFZ
Permoserstraße 15, 04318 Leipzig, Germany
Phone: +49 341 235 1784
pallav-kumar.shrestha@ufz.de
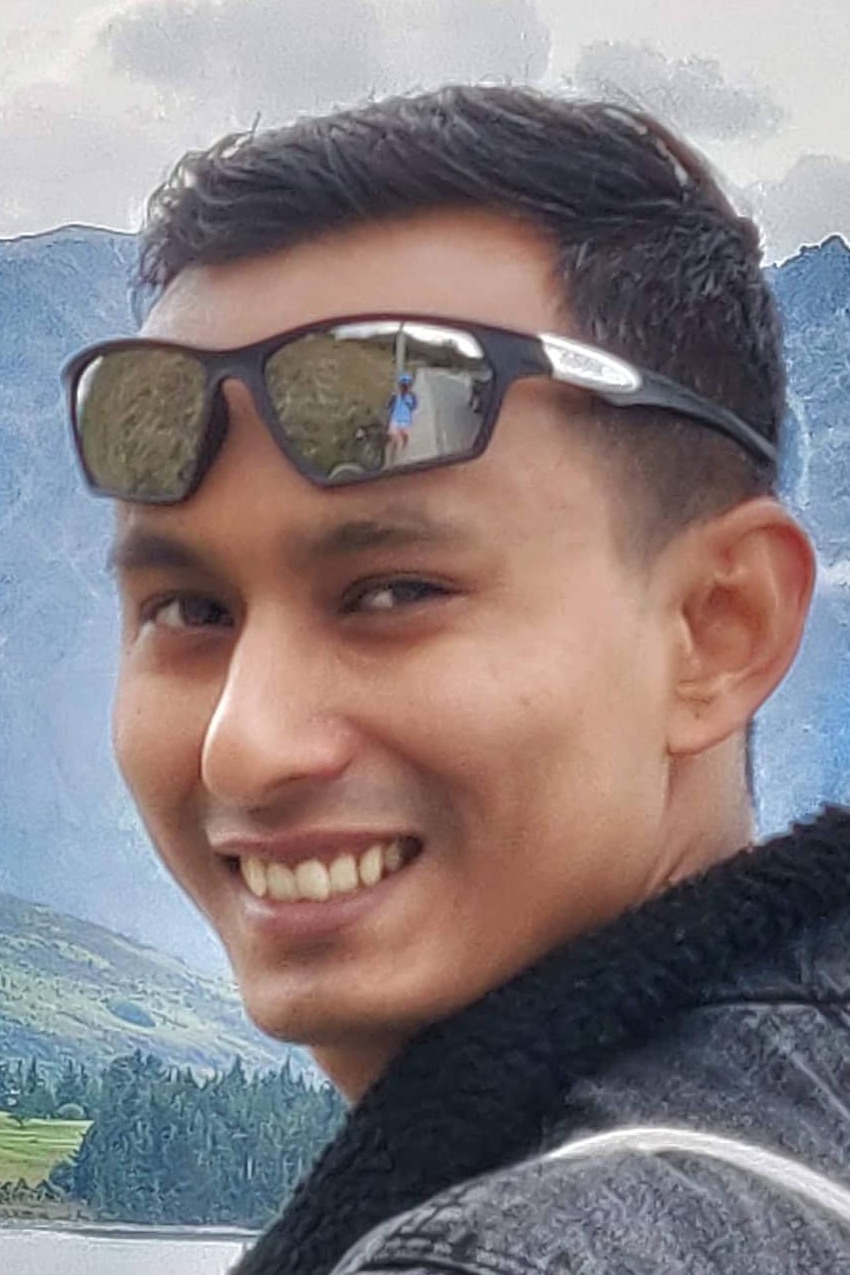
PhD Research Theme
The first chapter of my PhD is the development of a new reservoir module for mHM (published in WRR). The module automates the delineation of reservoirs and the catchments, eliminating the need for additional GIS preprocessing. The research involves three key aspects: 1) employing machine learning methods to reverse estimate non-consumptive demands (e.g., hydropower), 2) sensitivity of simulations to reservoir bathymetry, and 3) possible thresholds to identify and exclude non-disruptive reservoirs from the model simulation.
In the second chapter (submission preparation), we develop a new stream network upscaling technique called subgrid catchment conservation (SCC). Gridded hydrological models, such as mHM, incur simulation errors using the existing (classic or state-of-the-art) upscaling methods, compelling modelers to resort to sub-kilometer hyperresolution modeling. SCC offers three distinct advantages: 1) generates locally relevant streamflow, ensuring satisfactory model performance even at small gauge catchments (e.g., 1 sq. km.), irrespective of model resolution, 2) improves consistency of streamflow across model resolutions, and 3) resolves multiple gauges within a grid.
The third chapter (accepted in Nature Communications) is an application paper. We develop and test a high-resolution (1 km) flood early warning system, FEWS (with mHM), on a real flood event, retrospectively. This proof of concept produces impact forecast (e.g., affected buildings) as well as early notice time till maximum water level at each model grid.
The final chapter connects the two development papers and the application paper. We conduct analyses on flood attenuation through the introduction of hypothetical reservoirs upstream. Scenarios played out include number, size, location, and operation rules of the reservoir/s. Furthermore, we examine the sensitivity of mHM and, consequently, the FEWS to changes in model resolution. In essence, we assess the advantages of both coarse- and hyperresolution modeling within the FEWS framework, taking into consideration the implications of SCC.
Scientific Career
10/2017 - till date
PhD researcher
Department of Computational Hydrosystems (CHS) at UFZ, Leipzig, Germany
05/2014 - 10/2017
Water Resources Specialist and Programmer
Water Engineering and Management Program, Asian Institute of Technology (AIT), Thailand
2011 - 2013
Research Associate
Nepal Development and Research Institute, Kathmandu, Nepal
Lecturer
Kathamandu Engineering College, Tribhuvan University, Nepal
M.Sc. Water Resources Engineering
Institute of Engineering, Tribhuvan University, Nepal
Publications and Software
- Shrestha PK, Samaniego L, Rakovec O, Kumar R, Mi C, Rinke K, and Thober S (2024). Towards improved simulations of disruptive reservoirs in global hydrological modelling. Water Resources Research. https://doi.org/10.1029/2023WR035433
- Najafi H, Shrestha PK, Rakovec O, Apfel H, Vorogushyn S, Thober S, Kumar R, Merz B, and Samaniego L (2024, accepted). Advancing a High-Resolution Impact-based Early Warning System for Riverine Flooding. Nature Communications.
- Ogbu KN, Rakovec O, Shrestha PK, Samaniego L, Tischbein B, and Meresa H (2022). Testing the mHM-MPR Reliability for Parameter Transferability across Locations in North–Central Nigeria. Hydrology. https://doi.org/10.3390/hydrology9090158
- Saha TR, Shrestha PK, Rakovec O, Thober S and Samaniego L (2021). A drought monitoring tool for South Asia. Environmental Research Letters. https://doi.org/10.1088/1748-9326/abf525
- Bhatta B, Shrestha S, Shrestha PK and Talchabhadel R (2020). Modelling the impact of past and future climate scenarios on streamflow in a highly mountainous watershed: A case study in the West Seti River Basin, Nepal. Science of the Total Environment. https://doi.org/10.1016/j.scitotenv.2020.140156
- Mtilatila L, Bronstert A, Shrestha PK, Kadewere P and Vormoor K (2020). Susceptibility of Water Resources and Hydropower Production to Climate Change in the Tropics: The Case of Lake Malawi and Shire River Basins, SE Africa. Hydrology. https://doi.org/10.3390/hydrology7030054
- Shrestha M, Shrestha S and Shrestha PK (2019). Evaluation of landuse change and its impact on water yield in Songkhram River Basin, Thailand. International Journal of River Basin Management. https://doi.org/10.1080/15715124.2019.1566239
- Shrestha PK, Shrestha S and Ninsawat S (2018). How significant is sub‐daily variability of rainfall for hydrological modeling of floods? – A satellite based approach to sub‐daily downscaling of gauged rainfall. Meteorological Applications. https://doi.org/10.1002/met.1762
- Shrestha S, Hoang N, Shrestha, PK and Bhatta B (2018). Climate change impact on groundwater recharge and suggested adaptation strategies for selected Asian cities. APN Science Bulletin, 8(1). https://doi:10.30852/sb.2018.499
- Shrestha S, Bhatta B, Shrestha M and Shrestha PK (2018). Integrated assessment of the climate and landuse change impact on hydrology and water quality in the Songkhram River Basin, Thailand. Science of the Total Environment. https://doi.org/10.1016/j.scitotenv.2018.06.306
- Shrestha PK, Shakya NM, Pandey VP, Birkinshaw, SJ and Shrestha S (2017). Model-based Estimation of Land Subsidence in Kathmandu Valley, Nepal. Geomatics, Natural Hazard and Risk.
http://dx.doi.org/10.1080/19475705.2017.1289985
- Shrestha PK, Birkinshaw SJ and Shrestha, S (2017). Parallel execution with Automated Changes (PAC) module for SHETRAN. http://research.ncl.ac.uk/shetran/PAC.htm [Language: Linux bash]
- Shrestha M, Acharya S and Shrestha PK (2017). Bias Correction of climate models for hydrological modeling - are simple methods still useful? Meteorological Applications.
http://onlinelibrary.wiley.com/doi/10.1002/met.1655/full
- Shrestha S, Shrestha M and Shrestha PK (2017). Evaluation of SWAT models performance to simulate river discharge in Himalayan and tropical basins of Asia. Hydrology Research.
http://dx.doi.org/10.2166/nh.2017.189
- Shrestha PK (2016). SWAT Calibration Helper v1.0. VBA tailored Microsoft Excel Worksheet. Research Gate. https://doi.org/10.13140/RG.2.1.3760.1686 [Language: VBA]
- Aung MT, Shrestha S, Weesakul S and Shrestha PK (2016). Multi-model Climate Change Projections for Belu River Basin, Myanmar under Representative Concentration Pathways. Journal of Earth Science and Climate Change (7:1). http://dx.doi.org/10.4172/2157-7617.1000323
Conference Presentations
- Shrestha PK, Thober S, Samaniego L. Regionalization of Reservoir regulation parameters using physiographic and climatological predictors. European Geosciences Union General Assembly (EGU). 19 -– 30 Apr 2021, Online. https://meetingorganizer.copernicus.org/EGU21/EGU21-5030.html
- Shrestha PK, Thober S, Rakovec O, Najafi H, Lorenz C, Samaniego L. Impartially resolving reservoirs of all sizes for seamless hydrological forecasting using multiscale Lake Module (mLM). American Geophysical Union General Assembly (AGU). 7 -- 14 Dec 2020, Online. AGU abstract book - https://agu.confex.com/agu/fm20/meetingapp.cgi/Paper/695085
- Shrestha PK, Lorenz C, Najafi H, Thober S, Rakovec O, Samaniego L. Towards scale independent hydrological forecasting in regulated semi-arid regions. European Geosciences Union General Assembly (EGU). 4 -– 8 May 2020, Online. https://presentations.copernicus.org/EGU2020/EGU2020-6047_presentation.pdf
- Shrestha PK, Samaniego L, Rakovec O, Kumar R, Júnior FV, Martins E, and Thober S. Comprehensible modeling of reservoir regulation effect on streamflow with a scalable lake-hydrology model. American Geophysical Union General Assembly (AGU). 9 -– 13 Dec 2019, San Francisco. AGU abstract book - https://agu.confex.com/agu/fm19/meetingapp.cgi/Paper/534735
- Shrestha PK, Samaniego L, Thober S, Lorenz C, Behnia S, Portele TC, Laux P, Kumar R and Rakovec O. Towards Scale Independent Lake/Dam-hydrology Mod-elling in Seasonal Forecasting of Semi-arid Regions. International Union of Geodesy and Geophysics (IUGG) General Assembly. 8 - 18 Jul 2019, Montreal. IUGG abstract book - http://iugg2019montreal.com/assets/iugg2019-abstracts.zip
- Shrestha PK, Samaniego L, Thober S, Kumar R, Behnia S and Rakovec O. Towards scale independent lake-hydrology modeling in semi-arid regions: mHM lake module (mLM) development. European Geosciences Union (EGU) General Assembly. 7 - 12 Apr 2019, Vienna. https://meetingorganizer.copernicus.org/EGU2019/EGU2019-8054.pdf
- Shrestha PK, Samaniego L, Rakovec O, Thober S and Kumar R. Augmenting meso-scale hydrological model (mHM) for seasonal forecasting of lake-hydrology systems. European Geosciences Union (EGU) General Assembly. 8 - 13 Apr 2018, Vienna. https://meetingorganizer.copernicus.org/EGU2018/EGU2018-12940-1.pdf
- Shrestha PK, Shrestha S and Ninsawat S. Satellite based sub-daily downscaling of gauged rainfall for flood analysis via fully distributed hydrological model. THA2017 International Conference on Water Management and Climate Change towards Asias Water-Energy-Food Nexus. 25 - 27 Jan 2017, Bangkok.
- Shrestha PK, Shrestha S and Ninsawat S. Diurnal disaggregation of precipitation measurements with TRMM how good of an alternative is it to subdaily observations for distributed hydrological modeling? 1st Symposium on Tonle Sap Water Environment. 26 – 27 August, 2016. Phnom Penh, Cambodia.
- Shrestha PK, Shakya NM, Pandey VP, Birkinshaw SJ and Shrestha S. Model Based Land Subsidence in Kathmandu Valley, Nepal. International Conference on Resilience of Groundwater Systems to Climate Change and Human Development 8-11 February, 2016. Bangkok.
- Shrestha PK and Shrestha S. Multivariate Evaluation of a physically based Distributed Hydrological Model in Snow-fed River Basins of in Hindu Kush Himalaya Region. 2nd International Young Researchers Workshop on River Basin Environment and Management. 5 – 6 January, 2015, Hanoi.
- Shrestha D, Gyawali D, Basnyat DB and Shrestha PK. Bias Correction of Climate Change Projections on Koshi Basin. International conference on Climate Change, Water Resources and Disasters in Mountainous Regions: Building Resilience to Changing Climate. 27 – 29 Nov, 2013, Nepal.
- Shrestha PK, Shakya NM, Pandey VP, and Birkinshaw SJ. Distributed groundwater modeling for analysis of landslide hazard. 4th National Symposium on Challenges and opportunities for Sustainable Management of Groundwater Resources of Kathmandu Valley, Nepal, 22 st Mar, 2013, Nepal.