Dr. rer. nat. Falk Heße
Contact
Dr. rer. nat. Falk Heße
Department Computational Hydrosystems
Helmholtz-Centre for Environmental Sciences
Permoserstr. 15
04318 Leipzig, Germany
Tel: 0341 235 1050
falk.hesse@ufz.de
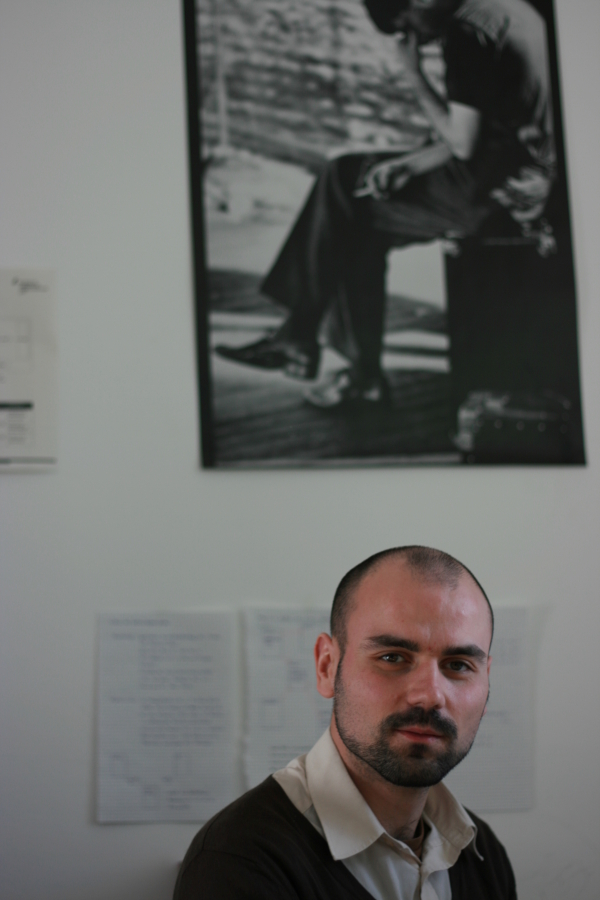
Scientific Career
since 2015
Postdoctoral Researcher at Helmholtzcentre for Environmental Science - UFZ, Department for Hydrosystems
2014
Visiting Scholar at the University of California at Berkeley, Department of Civil and Environmental Engineering
2012-2013
Postdoctoral Researcher at Helmholtzcentre for Environmental Science - UFZ, Department for Environmental Microbiology
2009-2012
Postdoctoral Researcher at the Friedrich-Schiller University Jena, Institute of Geosciences, Department of Hydrogeology
2006-2009
PhD Student at the Helmholtzcentre for Environmental Science - UFZ, Department
for Computational Hydrosystems
2003-2005
Master Student of Computational Sciences at the Technical University Chemnitz, Faculty of Natural Sciences, Department of Theoretical Physics
1999 - 2003
Diploma Student of Physical Engineering at the University of Applied Sciences Jena, Department SciTec Precision - Optics - Materials - Environment
Research interests
Multiscale Models, Numerics of Dynamical Systems, Stochastic Processes
Topics
Bayesian uncertainty characterization
The relevance of uncertainty analysis to hydrogeology is very high due to the combination of highly-heterogeneous subsurface properties and a general scarcity of available in-situ data. As a result, we need a general framework to represent and reason with uncertainty arising in the field of hydrogeology. Bayesian statistics provides a sound framework to that end. Compared to classic, frequentist, statistics, it can describe uncertainties more naturally, can handle single-case probabilities, which arise from in-situ site characterization, and is able to consistently transfer available background knowledge from ex-situ sites by virtue of the prior distribution.
However, both hydrogeological uncertainty analysis, and the inference used to that end, are rarely performed by using Bayesian methods. Arguably, the most important reason for this is the problem of prior derivation, i.e., the task of specifying the initial (un)certainty, prior to accounting for in-situ data. This lack has left the field of Bayesian uncertainty analysis incomplete, negatively affected the determination of data worth and hampered the adoption of Bayesian methods in general.
In this project, I want to address this problem by (i) establishing a workflow for hydrogeological prior derivation and (ii) assess the impact of these priors. For the first part, I will use the hierarchical model for prior derivation that has been developed in a collaboration of mine with the working group of Prof. Yoram Rubin. To provide this model with a representative and comprehensive dataset, I am going to couple it with a large database of hydrogeological measurements. This will facilitate the derivation highly-informative prior distributions that contain a significant amount of background knowledge derived from ex-situ data. In the second part, I am going to assess the impact of such informative priors on the question of uncertainty reduction and data worth for in-situ investigations. To that end, I will compare a series of classical analyzation tools to their analogous Bayesian counterpart. Emphasis will be put on the question of relative data worth, i.e., the impact of in-situ data compared to the already available ex-situ measurements.
The results of this project will help to establish a consistent and reproducible workflow of hydrogeological prior derivation, elucidate their resulting impact on the question of uncertainty reduction as well as the resulting relative data worth. Furthermore, it will help to better assess the advantages and drawbacks of Bayesian uncertainty analysis and determine challenges and possible problems that need to be addressed to make Bayesian statistics a general and widely-used framework for hydrogeological uncertainty analysis.
AquaDiva
The collaborated research centre (Sonderforschungsbereich) AquaDiva is dedicated to the investigation of how surface conditions and geology influence the functional biodiversity of the subsurface. Within this centre we are tasked with modelling the water and energy movement through the subsurface.
To that end, I apply the mesocsale Hydrological Model mHM, a spatially distributed hydrological model to the catchment of the AquaDiva observation site. Using detailed data of precipitation, land cover, morphology and soil type as inputs, mHM can determine fluxes like recharge and evapotranspiration and states like soil moisture as outputs.
Using these data, I apply the analytical framework developed by Botter et al. [2010, WRR] and Van der Velde et al. [2012, WRR] to quantify how the catchment stores and releases water. This allows for a description of the flow and transport dynamics taking place in the catchment. The spatial distribution of such dynamics can then be compared with land cover and soil moisture maps as well as driving forces like precipitation and temperature. Additionally I can determine the temporal evolution of mean travel times by using time series of all relevant hydrological processes from 1960-2010.
Teaching
Computational Fluid Dynamics: Magdeburg-Stendal University of Applied Sciences, International Master of Water Engineering
Mathematics and Modelling: Magdeburg-Stendal University of Applied Sciences, Master of Water Engineering
Modelling Flow and Transport in the Subsurface: Magdeburg-Stendal University of Applied Sciences, Master of Water Engineering