Working group
Land Cover / Dynamics (LACY)
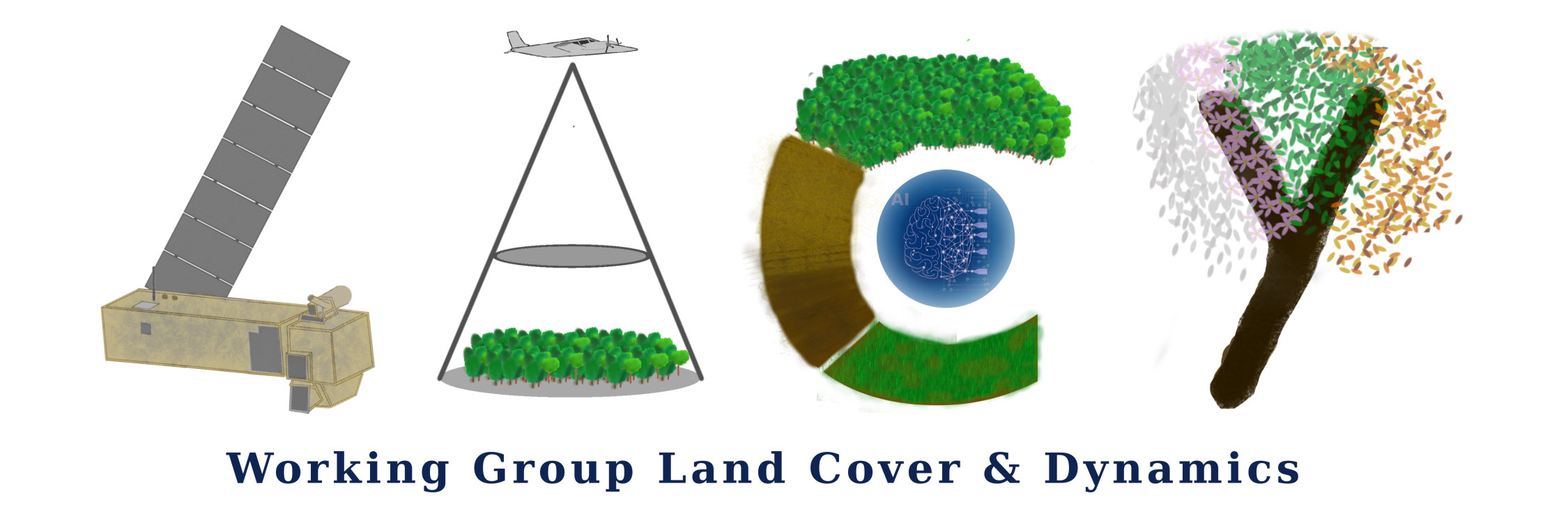
Short description
Our research focuses on the detection and evaluation of climate (extreme) effects and land-use / land-cover change on terrestrial vegetation. This concerns the following components:
1. Analysing optical-reflective time-series of satellite data to derive forest condition, plant traits (phenology, pigments, leaf area) and ecosystem services (biodiversity, productivity). This is done by inverse parameterisation of radiative transfer models, classical empirical approaches as well as data science methods.
2. Acquisition of remotely sensed hyperspectral (airborne and field) data accompanied by respective trait measurements and in-situ vegetation records
3. Derivation of land-use (crop types, tree species), land-use intensity (mowing events, grazing intensity, fertilisation) and habitats from optical-reflective time-series of satellite data using data science methods
4. Establish / evaluate links between land-use intensity / biodiversity and plant traits / vegetation condition
5. Design von processing chains of remotely sensed raw data
Forest Condition Anomalies
Share (%) of deciduous (left column) and non-deciduous (right column) forest area in Germany showing FCA values below −0.15 (strongly damaged or dead forest) in the years 2018 (top row) and 2022 (bottom row). Germany’s state borders are shown as black lines (Lange et al. 2024).
Biodiversity & its representation in remote sensing signals
General workflow from species and trait sampling, over grassland simulations and spectra generation to statistical analysis (Ludwig et al. 2024). Simulations were performed for five different diversity levels (5 to 25 species). Spectra were generated by passing the pixel-wise mean trait values to PROSAIL, for the same grassland simulation. Based on the pixel-wise reflectance values, spectral diversity was calculated. Finally, we calculated the correlation coefficients between the different spectral diversity metrics for Species Richness (SR), Shannon-Index, Simpson-Index and Rao’s Q.
Trait expressions on the relationship between spectral diversity and species richness (inspired by Diaz & Cabido, 2001).
Land-use intensity in grasslands
Methods and results deriving land-use intensity in grasslands (Lange et al. 2022).
Hyperspectral airborne campaigns
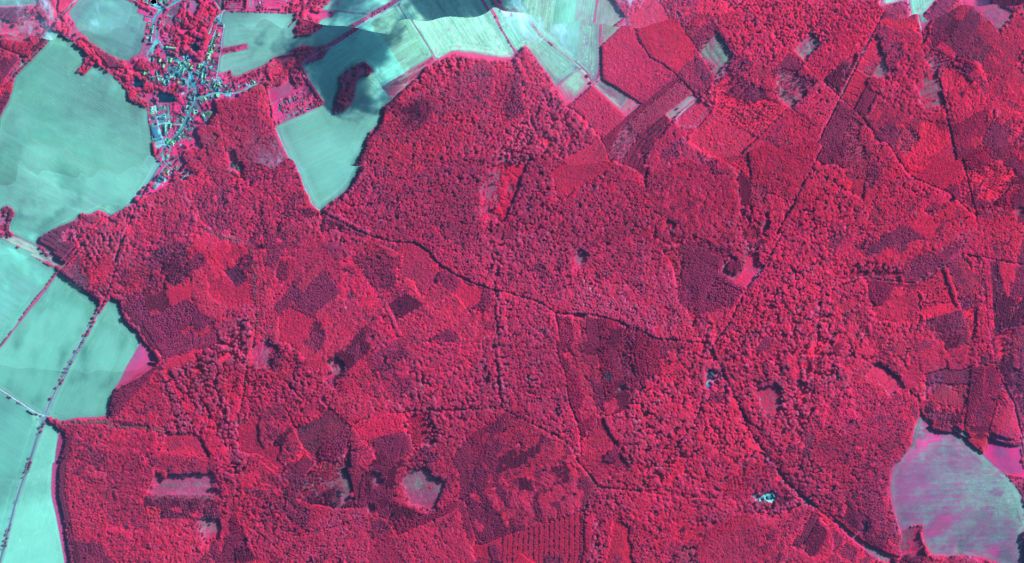
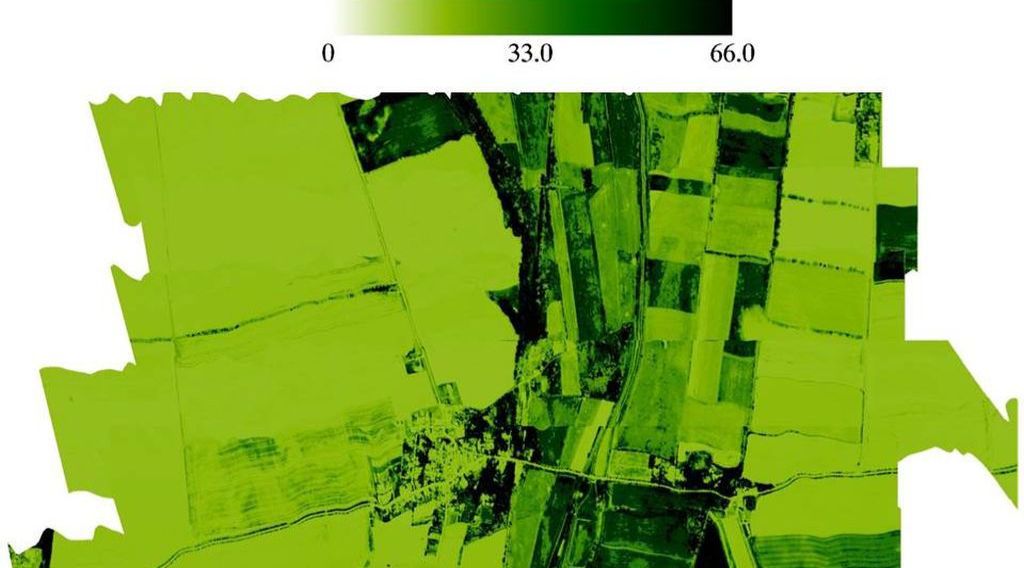
Extracting & simulating phenological metrics
The focus within vegetation phenology is on analysing the response of spring time phenology to climate change using ground and satellite observations. We also assessed the influence of heterogeneous landscapes on computed green-up dates and analysed trends of computed green-up dates on a European scale. A variety of methods to extract phenological metrics has been implemented in R package ’phenex’ to be of public use.
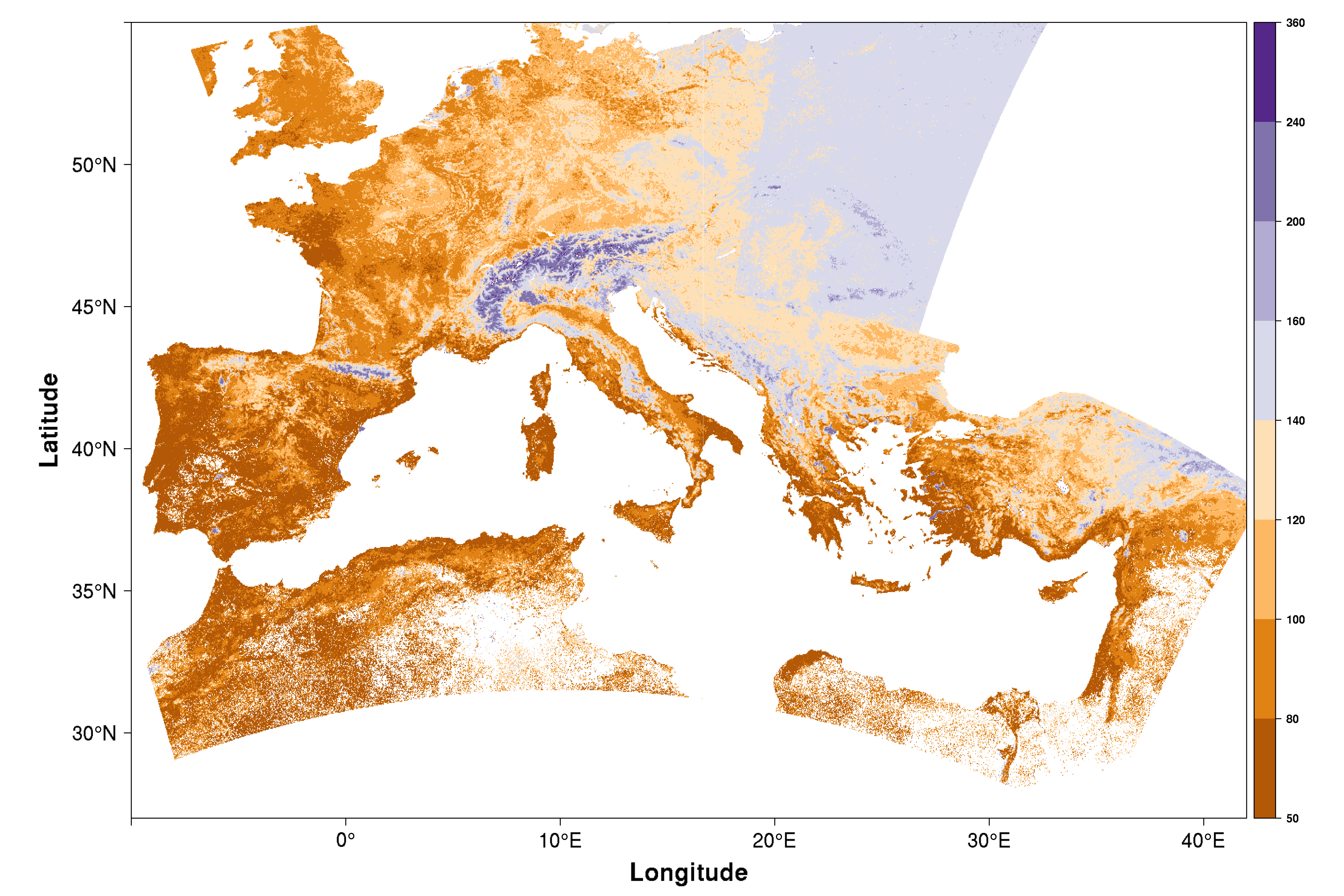
Extraction of biopyhsical vegetation variables / traits
The inversion of radiative transfer models is at the heart of determining e.g. Chlorophyll or water content of vegetation.
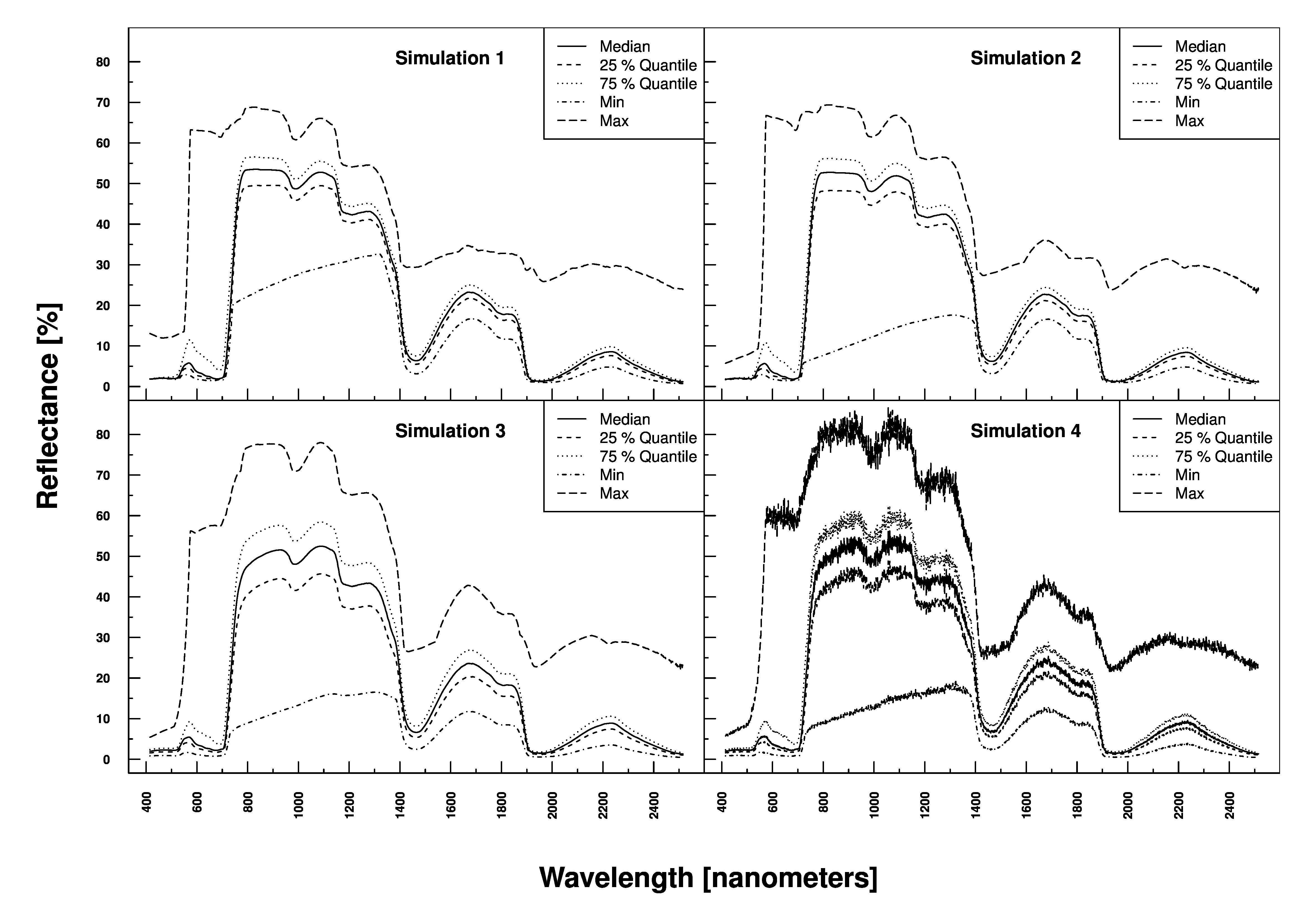
Land-use classification, habitat & biodiversity mapping
This is actually an old (remote sensing) topic which has seen a renaissance in the light of new satellite missions. This allows for example to discriminate tree species or to map crop types at field level (as shown below).
A WebGIS-based crop type map for the year 2016 can be found here.
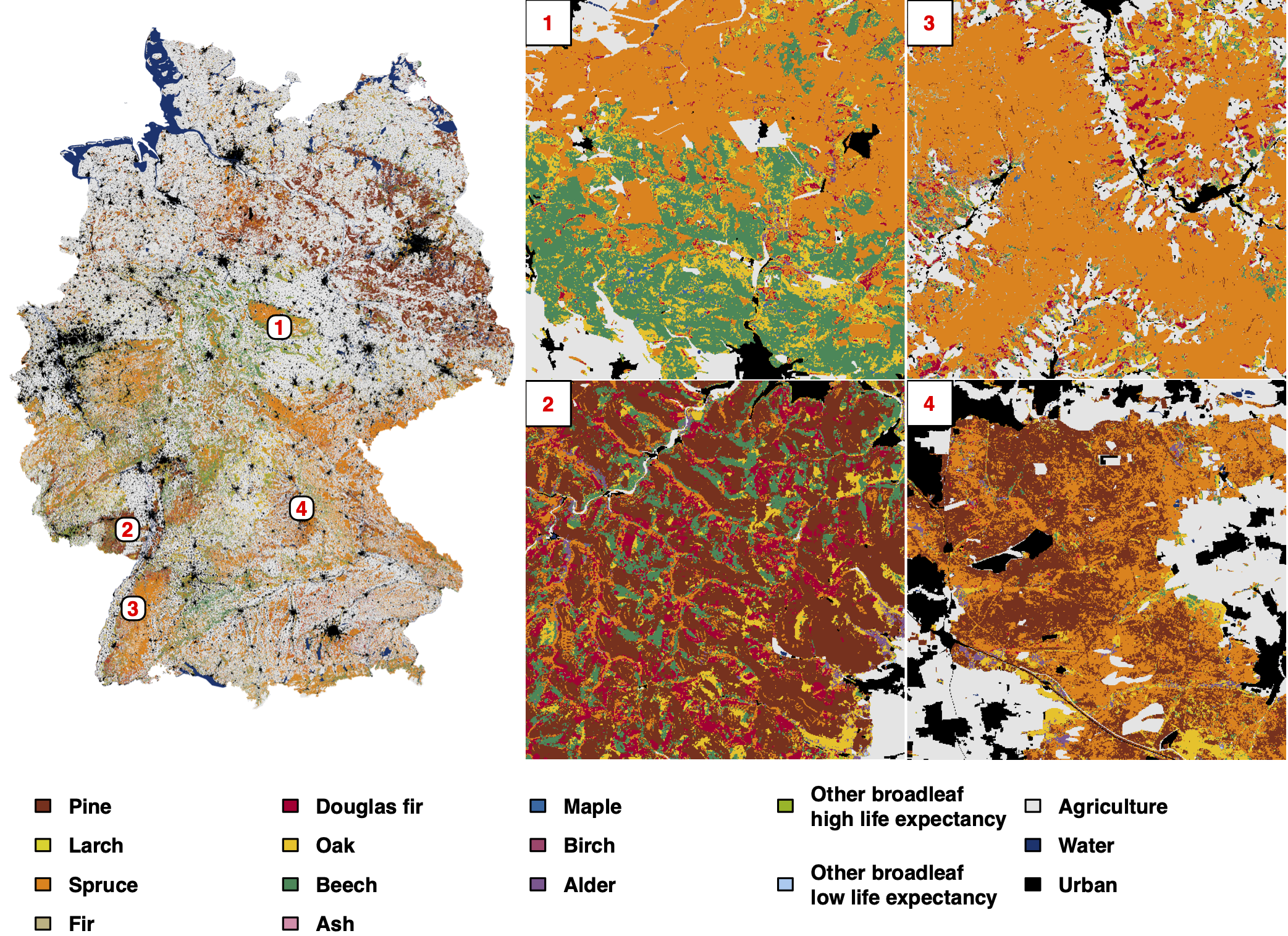
Contact:
- Time series analysis of multi-spectral satellite data (dynamic filtering, phenological metrics extraction)
- Radiative transfer modelling (PROSPECT, PROSAIL, SLC, DART) & inverse model parametrisation
- Neural networks (e.g. CNN), Machine learning (PCA, randomForest, PLSR, SVR, Gaussian processes)
- Field work: collecting spectral and in-situ plant trait data of grasslands, crops & forests
- Radiometric, geometric and atmospheric correction of hyper- and multispectral data
- High performance cluster computation
- Geostatistics (Kriging, Variogram estimation, MoransI)
- RSC4Earth, i.e. University of Leipzig (Faculty of Physics and Earth System Sciences)
- German Research Center for Geosciences, GFZ (Section "Remote Sensing")
- German Centre for Integrative Biodiversity Research (iDiv) Halle-Jena-Leipzig
- Julius-Kühn Institut (JKI), Institut für Strategien und Folgenabschätzung
- Thünen Institute of Forest Ecosystems
- University of Würzburg, Institute of Geography und Geology, Department of Remote Sensing
- German Aerospace Center (DLR), German Remote Sensing Data Center (DFD), Oberpfaffenhofen
- University of Zurich (Department of Geographie, Remote Sensing)
- HU Berlin (Institute of Geography, Geomatik)
- FH Dessau (Geoinformation)
Ongoing
- "Impacts of hydroclimatic extremes on long- term forest condition anomalies" in PhD cohort "Societal and Environmental impacts of complex ExtremeS in a chAnging World" (Helmholtz Association, 2025-2029)
- "Naturschutzfachliche bundesweite Waldpotenzialkarte für die vorgelagerte Planung - Nawapoka" (BfN, 2023-2026), PI
- "Satellitengestützte Aufnahme wesentlicher Waldökosystemfunktionen (Resilienzfaktoren) und Herleitung eines Bewertungsrahmens" (BMEL, 2024-2025)
- Pixel-spezifische Parametrisierung von Modellen zur Ableitung von Pflanzenzustandsvariablen aus Hyperspektraldaten und zur Ertragsabschätzung - Hy-PiPE (BMVI, 2022-2025)
Finished
- 'Forest Condition Monitor Germany' (Helmholtz Association, 2021-2024), PI
- "Towards robust detection of plant diversity & management in grasslands’ spectral signal" (iDiv flexpool, 2022-2024), PI
- "KI-basierte Integration von Fernerkundungs- und Citizen-Science- Daten zur Ableitung der Biodiversität in Wäldern’, iForest" (BMBF, 2023-2024), PI
- „Ermittlung naturschutzbezogener Kriterien in der Umweltprüfung der Bedarfsplanung für Stromnetze und in der Bundesfachplanung zur Erhöhung der Planungssicherheit und Verhinderung von Zielkonflikten“ - Wakanaka (BfN, 2017-2019)
- EU 'Ecopotential' ('Horizon 2020'). 'Derivation of bio-physical variables from remotely sensed imagery' (2015-2018)
- Helmholtz-Alliance ‘Remote Sensing and Earth System Dynamics’ (Biosphere): 'Fusion of radar (L-band) and hyperspectral data to derive biomass, leaf area index and vegetation disturbance' (2012-2017)
- BMWi program ‚Vorbereitung der wissenschaftlichen und kommerziellen Nutzung der Sentinel-Missionen und nationalen Missionen‘: ‚PhenoS - Phänologische Strukturierung von zeitlich hochauflösenden Sentinel 2- Datensätzen zur Optimierung von Landnutzungsklassifikationen‘ (2013- 2016)
- BfN assignement on ‚Characterisation of forest types using remotely sensed imagery‘ (2016)
- BMWi program ‚Vorbereitung der wissenschaftlichen und kommerziellen Nutzung der Sentinel-Missionen und nationalen Missionen‘: ‘Validierung von Sentinel-Produkten auf Basis kontinuierlicher spektraler und Eddy- Flux-Messungen’ (2012-2015)
- BMWi program ‚Development of methods and algorithms for data analysis in preparation of the EnMAP mission': ‘Methoden zur Ableitung des funktionellen Zusammenhanges ökosystemarer Prozesse in hyperspektralen Daten unterschiedlicher räumlicher Auflösung’ (2010- 2013)
- Project within ‚Ecosystem Services under Changing Land-use and Climate‘ (ESCALATE, http://www.ufz.de/escalate/): Ecosystem services assessment in a Central European floodplain forest: an ecosystem approach using reflective and thermal remote sensing data (2013-2016)
- WP 3 within the BMBF Projekt ‚Sustainable Land and Water Management of Reservoir Catchments‘ (SaLMaR, http://salmar.uni- jena.de/7659.0.html? &L=2): ‚Remote sensing of land use land cover (LULC) and point sources‘ (2012-2015)
Integrated projects (IP, UFZ fundet):
1) Within the topic 'From local scale processes to regional predictions' (T53) => „Estimation of terrestrial gross primary production (GPP) from remote sensing data“ (2013-2016 and 2016-2019)
2) Within ‚Catchment Dynamics‘ (T31) =>“Estimating organic carbon and plant properties from remotely sensed data“ (2013-2016)
Index:
- 2025 (4)
- 2024 (7)
- 2023 (1)
- 2022 (3)
- 2021 (1)
- 2020 (4)
- 2018 (1)
- 2017 (5)
- 2016 (6)
- 2015 (3)
- 2014 (4)
- 2013 (6)
- 2012 (1)
- 2011 (1)
- 2009 (1)
You could use our publication index for further requests.
2025 (4)
- Iakunin, M., Goss, R., Doktor, D. (2025):
Grassland management and phenology affect trait retrieval accuracy from remote sensing observations - field campaign dataset
Zenodo 10.5281/zenodo.14777369 - Iakunin, M., Taubert, F., Goss, R., Sasso, S., Feilhauer, H., Doktor, D. (2025):
Grassland management and phenology affect trait retrieval accuracy from remote sensing observations
Ecol. Inform. 87 , art. 103068 10.1016/j.ecoinf.2025.103068 - Reichmuth, A., Kühn, I., Schmidt, A., Doktor, D. (2025):
Forested Natura 2000 sites under climate change: effects of tree species distribution shifts
Web Ecol. 25 (1), 59 - 89 10.5194/we-25-59-2025 - Reichmuth, A., Rakovec, O., Boeing, F., Müller, S., Samaniego, L., Marx, A., Komischke, H., Schmidt, A., Doktor, D. (2025):
BioVars - A bioclimatic dataset for Europe based on a large regional climate ensemble for periods in 1971–2098
Sci. Data 12 , art. 217 10.1038/s41597-025-04507-w
2024 (7)
- Lange, M., Preidl, S., Reichmuth, A., Heurich, M., Doktor, D. (2024):
A continuous tree species-specific reflectance anomaly index reveals declining forest condition between 2016 and 2022 in Germany
Remote Sens. Environ. 312 , art. 114323 10.1016/j.rse.2024.114323 - Lange, M., Preidl, S., Reichmuth, A., Heurich, M., Doktor, D. (2024):
Forest condition anomaly index values covering Germany for 2016-2023 (7.5.0) [Data set]
Zenodo 10.5281/zenodo.13123397 - Ludwig, A., Doktor, D., Feilhauer, H. (2024):
Is spectral pixel-to-pixel variation a reliable indicator of grassland biodiversity? A systematic assessment of the spectral variation hypothesis using spatial simulation experiments
Remote Sens. Environ. 302 , art. 113988 10.1016/j.rse.2023.113988 - Mahecha, M.D., Bastos, A., Bohn, F.J., Eisenhauer, N., Feilhauer, H., Hickler, T., Kalesse-Los, H., Migliavacca, M., Otto, F.E.L., Peng, J., Sippel, S., Tegen, I., Weigelt, A., Wendisch, M., Wirth, C., Al-Halbouni, D., Deneke, H.M., Doktor, D., Dunker, S., Duveiller, G., Ehrlich, A., Foth, A., García-García, A., Guerra, C.A., Guimarães- Steinicke, C., Hartmann, H., Henning, S., Herrmann, H., Hu, P., Ji, C., Kattenborn, T., Kolleck, N., Kretschmer, M., Kühn, I., Luttkus, M.L., Maahn, M., Mönks, M., Mora, K., Pöhlker, M., Reichstein, M., Rüger, N., Sánchez-Parra, B., Schäfer, M., Stratmann, F., Tesche, M., Wehner, B., Wieneke, S., Winkler, A.J., Wolf, S., Zaehle, S., Zscheischler, J., Quaas, J. (2024):
Biodiversity and climate extremes: known interactions and research gaps
Earth Future 12 (6), e2023EF003963 10.1029/2023EF003963 - Meshkini, K., Bovolo, F., Doktor, D. (2024):
Attention-based 3D convolutional neural network for crop boundary detection in high-resolution satellite image time series
In: Bruzzone, L., Bovolo, F. (eds.)
Artificial Intelligence and Image and Signal Processing for Remote Sensing XXX 2024, Edinburgh, 16-18 September 2024
Proceedings / SPIE 13196
SPIE, Bellingham, WA, p. 131960E 10.1117/12.3035893 - Reichmuth, A. (2024):
Forested Natura 2000 sites under climate change
Version: N2K_2.1 Zenodo 10.5281/zenodo.11400444 - Reichmuth, A., Rakovec, O., Boeing, F., Müller, S., Samaniego, L., Marx, A., Komischke, H., Doktor, D. (2024):
BioVars - bioclimatic datasets for Europe based on a large regional climate ensemble for periods between 1971 to 2098 (1.1.0) [Data set]
Zenodo 10.5281/zenodo.14624171
2023 (1)
- Borrmann, P., Brandt, P., Gerighausen, H. (2023):
MISPEL: A multi-crop spectral library for statistical crop trait retrieval and agricultural monitoring
Remote Sens. 15 (14), art. 3664 10.3390/rs15143664
2022 (3)
- Hase, N., Doktor, D., Rebmann, C., Dechant, B., Mollenhauer, H., Cuntz, M. (2022):
Identifying the main drivers of the seasonal decline of near-infrared reflectance of a temperate deciduous forest
Agric. For. Meteorol. 313 , art. 108746 10.1016/j.agrformet.2021.108746 - Lange, M., Feilhauer, H., Kühn, I., Doktor, D. (2022):
Mapping land-use intensity of grasslands in Germany with machine learning and Sentinel-2 time series
Remote Sens. Environ. 277 , art. 112888 10.1016/j.rse.2022.112888 - Ludwig, A.D., Doktor, D., Goss, R., Sasso, S., Feilhauer, H. (2022):
The leaf is always greener on the other side of the lab: Optical in-situ indicators for leaf chlorophyll content need improvement for semi-natural grassland areas
Ecol. Indic. 143 , art. 109424 10.1016/j.ecolind.2022.109424
2021 (1)
- Feilhauer, H., Zlinszky, A., Kania, A., Foody, G.M., Doktor, D., Lausch, A., Schmidtlein, S. (2021):
Let your maps be fuzzy!—Class probabilities and floristic gradients as alternatives to crisp mapping for remote sensing of vegetation
Remote Sens. Ecol. Conserv. 7 (2), 292 - 305 10.1002/rse2.188
2020 (4)
- Arnold, R., Haug, J.-K., Lange, M., Friesen, J. (2020):
Impact of forest cover change on available water resources: Long-term forest cover dynamics of the semi-arid Dhofar cloud forest, Oman
Front. Earth Sci. 8 , art. 299 10.3389/feart.2020.00299 - Ayalew, D.A., Deumlich, D., Šarapatka, B., Doktor, D. (2020):
Quantifying the sensitivity of NDVI-based C factor estimation and potential soil erosion prediction using spaceborne Earth observation data
Remote Sens. 12 (7), art. 1136 10.3390/rs12071136 - Kattge, J., Bönisch, G., Díaz, S., Lavorel, S., Beckmann, M., Dechant, B., Durka, W., Klotz, S., Roscher, C., Doktor, D., Prentice, I.C., et al. (2020):
TRY plant trait database – enhanced coverage and open access
Glob. Change Biol. 26 (1), 119 - 188 10.1111/gcb.14904 - Preidl, S., Lange, M., Doktor, D. (2020):
Introducing APiC for regionalised land cover mapping on the national scale using Sentinel-2A imagery
Remote Sens. Environ. 240 , art. 111673 10.1016/j.rse.2020.111673
2018 (1)
- Rocchini, D., Luque, S., Pettorelli, N., Bastin, L., Doktor, D., Faedi, N., Feilhauer, H., Féret, J.-B., Foody, G.M., Gavish, Y., Godinho, S., Kunin, W.E., Lausch, A., Leitão, P.J., Marcantonio, M., Neteler, M., Ricotta, C., Schmidtlein, S., Vihervaara, P., Wegmann, M., Nagendra, H. (2018):
Measuring β-diversity by remote sensing: A challenge for biodiversity monitoring
Methods Ecol. Evol. 9 (8), 1787 - 1798 10.1111/2041-210X.12941
2017 (5)
- Dechant, B., Cuntz, M., Vohland, M., Schulz, E., Doktor, D. (2017):
Estimation of photosynthesis traits from leaf reflectance spectra: Correlation to nitrogen content as the dominant mechanism
Remote Sens. Environ. 196 , 279 - 292 10.1016/j.rse.2017.05.019 - Lange, M., Dechant, B., Rebmann, C., Vohland, M., Cuntz, M., Doktor, D. (2017):
Validating MODIS and Sentinel-2 NDVI products at a temperate deciduous forest site using two independent ground-based sensors
Sensors 17 (8), art. 1855 10.3390/s17081855 - Padró, J.-C., Pons, X., Aragonés, D., Díaz-Delgado, R., García, D., Bustamante, J., Pesquer, L., Domingo‐Marimon, C., González-Guerrero, O., Cristóbal, J., Doktor, D., Lange, M. (2017):
Radiometric correction of simultaneously acquired Landsat-7/Landsat-8 and Sentinel-2A imagery using Pseudoinvariant Areas (PIA): Contributing to the Landsat time series legacy
Remote Sens. 9 (12), art. 1319 10.3390/rs9121319 - Schröter, I., Paasche, H., Doktor, D., Xu, X., Dietrich, P., Wollschläger, U. (2017):
Estimating soil moisture patterns with remote sensing and terrain data at the small catchment scale
Vadose Zone J. 16 (10) 10.2136/vzj2017.01.0012 - Xu, X., Conrad, C., Doktor, D. (2017):
Optimising phenological metrics extraction for different crop types in Germany using the Moderate Resolution Imaging Spectrometer (MODIS)
Remote Sens. 9 (3), art. 254 10.3390/rs9030254
2016 (6)
- Carl, G., Doktor, D., Schweiger, O., Kühn, I. (2016):
Assessing relative variable importance across different spatial scales: a two-dimensional wavelet analysis
J. Biogeogr. 43 (12), 2502 - 2512 10.1111/jbi.12781 - Feilhauer, H., Doktor, D., Schmidtlein, S., Skidmore, A.K. (2016):
Mapping pollination types with remote sensing
J. Veg. Sci. 27 (5), 999 - 1011 10.1111/jvs.12421 - Gerstmann, H., Doktor, D., Gläßer, C., Möller, M. (2016):
PHASE: A geostatistical model for the Kriging-based spatial prediction of crop phenology using public phenological and climatological observations
Comput. Electron. Agric. 127 , 726 - 738 10.1016/j.compag.2016.07.032 - Lange, M., Schaber, J., Marx, A., Jäckel, G., Badeck, F.W., Seppelt, R., Doktor, D. (2016):
Simulation of forest tree species’ bud burst dates for different climate scenarios: chilling requirements and photo-period may limit bud burst advancement
Int. J. Biometeorol. 60 (11), 1711 - 1726 10.1007/s00484-016-1161-8 - Luft, L., Neumann, C., Itzerott, S., Lausch, A., Doktor, D., Freude, M., Blaum, N., Jeltsch, F. (2016):
Digital and real-habitat modeling of Hipparchia statilinus based on hyper spectral remote sensing data
Int. J. Environ. Sci. Technol. 13 (1), 187 - 200 10.1007/s13762-015-0859-1 - Richter, R., Reu, B., Wirth, C., Doktor, D., Vohland, M. (2016):
The use of airborne hyperspectral data for tree species classification in a species-rich Central European forest area
Int. J. Appl. Earth Obs. Geoinf. 52 , 464 - 474 10.1016/j.jag.2016.07.018
2015 (3)
- Lausch, A., Salbach, C., Schmidt, A., Doktor, D., Merbach, I., Pause, M. (2015):
Deriving phenology of barley with imaging hyperspectral remote sensing
Ecol. Model. 295 , 123 - 135 10.1016/j.ecolmodel.2014.10.001 - Neumann, C., Weiss, G., Schmidtlein, S., Itzerott, S., Lausch, A., Doktor, D., Brell, M. (2015):
Gradient-based assessment of habitat quality for spectral ecosystem monitoring
Remote Sens. 7 (3), 2871 - 2898 10.3390/rs70302871 - Paz-Kagan, T., Zaady, E., Salbach, C., Schmidt, A., Lausch, A., Zacharias, S., Notesco, G., Ben-Dor, E., Karnieli, A. (2015):
Mapping the spectral soil quality index (SSQI) using airborne imaging spectroscopy
Remote Sens. 7 (11), 15748 - 15781 10.3390/rs71115748
2014 (4)
- Brosinsky, A., Lausch, A., Doktor, D., Salbach, C., Merbach, I., Gwillym-Margianto, S., Pause, M. (2014):
Analysis of spectral vegetation signal characteristics as a function of soil moisture conditions using hyperspectral remote sensing
J. Indian Soc. Remote Sens. 42 (2), 311 - 324 10.1007/s12524-013-0298-8 - Doktor, D., Lausch, A., Spengler, D., Thurner, M. (2014):
Extraction of plant physiological status from hyperspectral signatures using machine learning methods
Remote Sens. 6 (12), 12247 - 12274 10.3390/rs61212247 - Feilhauer, H., Dahlke, C., Doktor, D., Lausch, A., Schmidtlein, S., Schulz, G., Stenzel, S. (2014):
Mapping the local variability of Natura 2000 habitats with remote sensing
Appl. Veg. Sci. 17 (4), 765 - 779 10.1111/avsc.12115 - Wollschläger, U., Grau, T., Martini, E., Neubauer, M., Schmidt, A., Schrön, M., Schröter, I., Dietrich, P., Fleckenstein, J.H., Lausch, A., Musolff, A., Paasche, H., Reinstorf, F., Vogel, H.-J., Werban, U., Zacharias, S. (2014):
Revealing principles of hydrological response – the Schaefertal approach
Proceedings of the 2014 AGU Chapman Conference on Catchment Spatial Organization and Complex Behavior, Luxembourg, 23-26. September, 2014
1 - 3
2013 (6)
- Brell, M., Rogass, C., Segl, K., Spengler, D., Kuester, T., Itzerott, S., Chabrillat, S., Roessner, S., Lausch, A., Doktor, D., Kaufmann, H. (2013):
An automated pre-processing chain for airborne hyperspectral data including radiometric calibration, atmospheric correction and direct georeferencing
Conference: 8th EARSeL SIG Imaging Spectroscopy Workshop Nantes, France
10.13140/2.1.2932.1604 - Carl, G., Doktor, D., Koslowsky, D., Kühn, I. (2013):
Phase difference analysis of temperature and vegetation phenology for beech forest: a wavelet approach
Stoch. Environ. Res. Risk Assess. 27 (5), 1221 - 1230 10.1007/s00477-012-0658-x - Lausch, A., Pause, M., Doktor, D., Preidl, S., Schulz, K. (2013):
Monitoring and assessing of landscape heterogeneity at different scales
Environ. Monit. Assess. 185 (11), 9419 - 9434 10.1007/s10661-013-3262-8 - Lausch, A., Pause, M., Merbach, I., Zacharias, S., Doktor, D., Volk, M., Seppelt, R. (2013):
A new multiscale approach for monitoring vegetation using remote sensing-based indicators in laboratory, field, and landscape
Environ. Monit. Assess. 185 (2), 1215 - 1235 10.1007/s10661-012-2627-8 - Lausch, A., Pause, M., Schmidt, A., Salbach, C., Gwillym-Margianto, S., Merbach, I. (2013):
Temporal hyperspectral monitoring of chlorophyll, LAI and water content of barley during a growing season
Can. J. Remote Sens. 39 (3), 191 - 207 10.5589/m13-028 - Lausch, A., Zacharias, S., Dierke, C., Pause, M., Kühn, I., Doktor, D., Dietrich, P., Werban, U. (2013):
Analysis of vegetation and soil patterns using hyperspectral remote sensing, EMI, and gamma-ray measurements
Vadose Zone J. 12 (4) 10.2136/vzj2012.0217
2012 (1)
- Doktor, D., Lange, M., Koslowsky, D. (2012):
Evaluation of methods to compute green-up dates based on daily NDVI observations
2012 IEEE 32nd International Geoscience and Remote Sensing Symposium, Munich, Germany, 22-27 July 2012
International Geoscience and Remote Sensing Symposium IGARSS2012
Institute of Electrical and Electronics Engineers (IEEE), New York, NY, p. 4922 - 4925 10.1109/IGARSS.2012.6352508
2011 (1)
- Rogass, C., Spengler, D., Bochow, M., Segl, K., Lausch, A., Doktor, D., Roessner, S., Behling, R., Wetzel, H.-U., Kaufmann, H. (2011):
Reduction of radiometric miscalibration-applications to pushbroom sensors
Sensors 11 (6), 6370 - 6395 10.3390/s110606370
2009 (1)
- Doktor, D., Bondeau, A., Koslowski, D., Badeck, F.-W. (2009):
Influence of heterogeneous landscapes on computed green-up dates based on daily AVHRR NDVI observations
Remote Sens. Environ. 113 (12), 2618 - 2632 10.1016/j.rse.2009.07.020