Working group
Terrestrial Water Dynamics (TWD)
Research focus
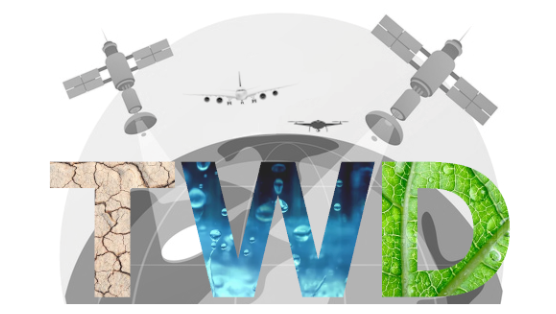
The group aims to quantify terrestrial water cycle dynamics from the integration of multi-source Earth observations and modelling framework across scales. Another focus lies on the investigation of land-atmosphere interaction and climate extremes using novel remote sensing products (e.g., detection of extreme events, impacts of extremes on water cycle and ecosystems). In addition, we also explore machine learning methods to improve monitoring and modelling performance (e.g., fusing and downscaling multi-source remote sensing products, uncertainty quantification, hybrid modelling).
Group members
Research activities
Satellite-based large-scale water and energy fluxes
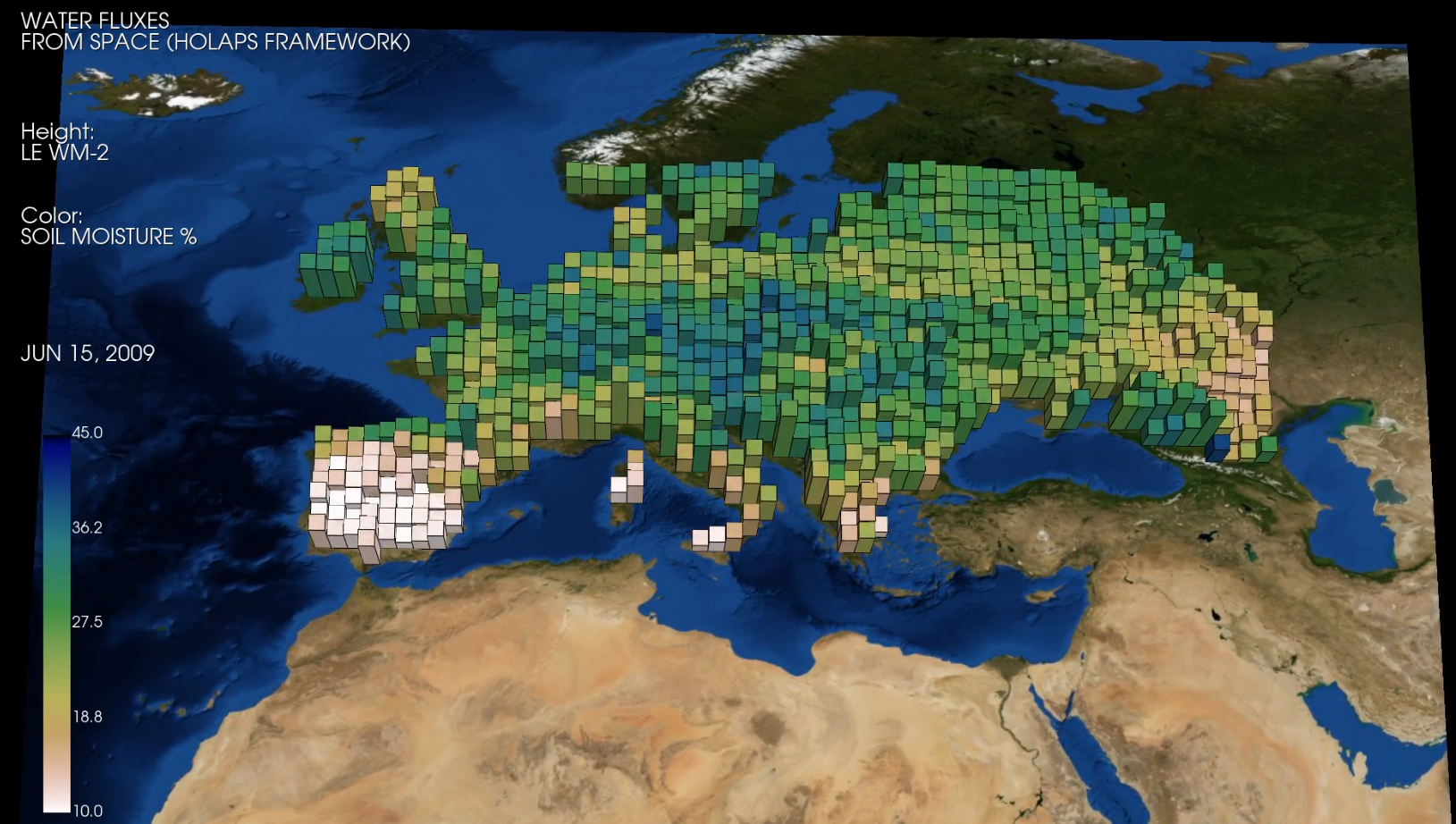
The energy and water exchanges between the land surface and the lower atmosphere are a key driver of the Earth’s climate system. We are working to provide physically consistent estimates of energy and water fluxes at the land surface from regional to global scales at high temporal and spatial resolution. The main tool is the High resOlution Land Atmosphere surface Parameters from Space (HOLAPS) framework, which is able to derive surface fluxes from satellite remote sensing observations. The final products will be publicly available and could be used for different applications, such as large- and regional-scale hydrological and agricultural analyses, climate studies, research on land-atmosphere interactions, development and evaluation of weather and climate models.
Global Ground Heat Flux Estimation and Trend Analysis
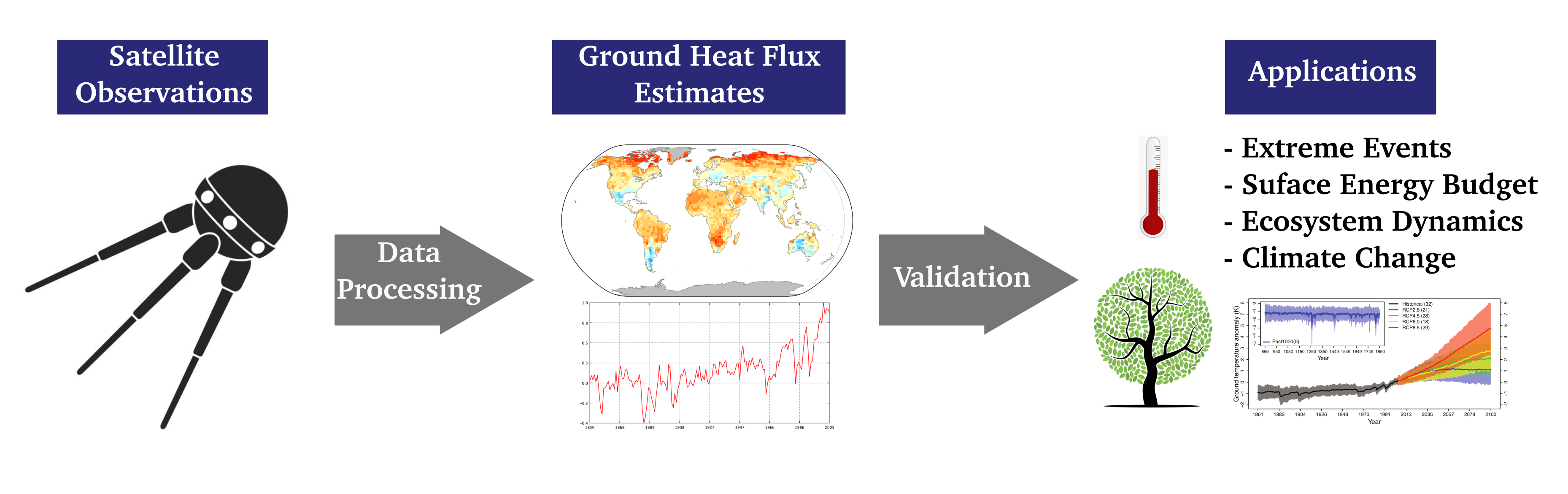
Accurate estimation of ground heat flux is crucial for ensuring the closure of the surface energy balance. Changes in ground heat flux are the main mechanism increasing the heat storage within the continental subsurface. Continental heat storage is the second largest term of the Earth’s energy inventory, that is, the additional energy gained by the Earth system due to the Earth’s energy imbalance. Despite its importance for understanding climate change, our knowledge about the magnitude of and changes in ground heat flux is critically hindered by the markedly scarce measurements available and its uneven geographical distribution. This project uses satellite remote sensing observations to derive a global, high resolution estimate of ground heat flux, in order to enhance our understanding of the surface energy balance and the land-atmosphere coupling. Furthermore, this estimate of ground heat flux may bridge the gap between paleoreconstructions of continental heat storage and current micrometeorological observations, expanding our knowledge about the mechanisms governing past and current climate change. More details can be found here.
Time series of fine-resolution surface albedo from coupling multisource satellite observations
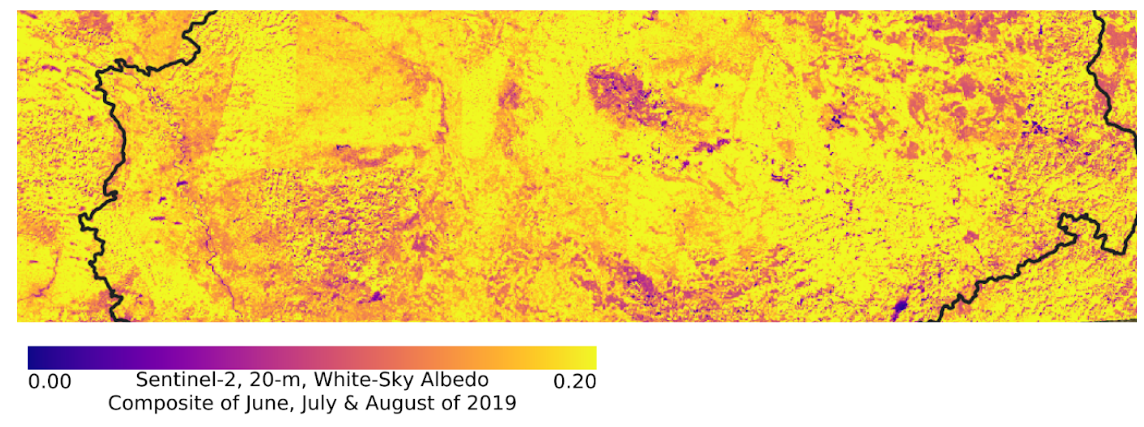
Surface albedo is an essential climate variable (ECV), required to monitor and model the surface energy, and the hydrologic and biogeochemical functioning of land and coastal ecosystems over the globe. The dilemma for satellite-based albedo retrievals is that the requirements of both fine spatial resolution and sufficient multi-angle observations (for retrievals of surface anisotropy to establish true albedo quantities) cannot be simultaneously fulfilled by current space-based sensors. Images at fine spatial resolutions, such as captured by Landsat-8 and Sentinel-2, provides excellent information on surface heterogeneity, but cannot provide adequate multi-angle observations to accurately capture surface anisotropy (i.e., bidirectional reflectance distribution function, BRDF) and produce realistic bihemispherical albedo quantities. This anisotropy information can, however, be derived from a multi-day accumulation of multi-angle observations from wide-swath and coarser-resolution imagers such as MODIS and VIIRS. In this research activity, we aim to produce time series of surface albedo at 20-m resolution by coupling the surface reflectances from the Sentinel-2 satellites with surface anisotropy information (as described by BRDF) from either the MODIS or VIIRS.
Contact: jian.peng@ufz.de
SAR-based high-resolution soil moisture retrieval
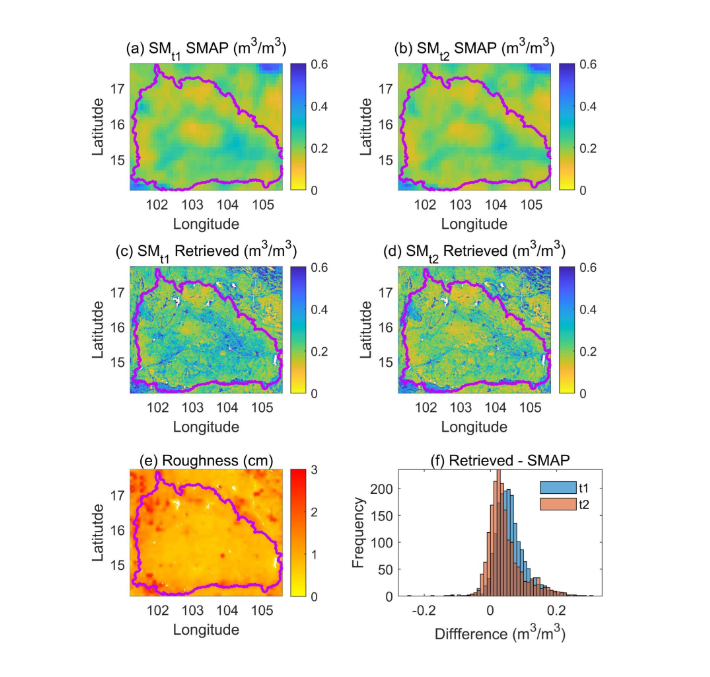
Soil moisture is an essential component of the Earth system and plays a critical role in the exchange of water, energy, and biogeochemical fluxes between the land surface and the atmosphere. Microwave remote sensing provides valuable observations for the estimation of surface soil moisture. In this project, we aim to provide high-resolution soil moisture products at regional and global scales. Our main method is the dual-temporal dual-channel (DTDC) algorithm, which can estimate the land surface soil moisture by using time-series observations from the Sentinel-1 C-band synthetic aperture radar (SAR). The developed soil moisture products are supposed to support applications such as drought analysis, numerical weather prediction, hydrometeorological disasters monitoring.
Contact: jian.peng@ufz.de
High-resolution soil moisture estimation from the synergy of multi-source data
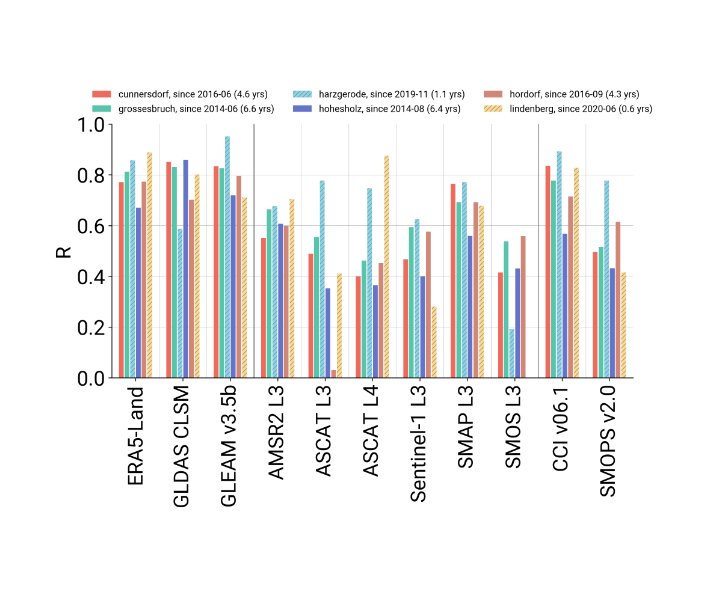
Current space-borne microwave-based soil moisture products are normally limited to single sensors and are at coarse spatial resolution, while high-resolution estimations are beneficial for numerous applications, like drought/flood predictions, precision agriculture, numerical weather prediction, or climate modeling. Thus we work on a synergistic data assimilation framework which can integrate multi-source data with prior information and radiative transfer models. Satellite data from different sensors in microwave, optical, and thermal domains, like Sentinel-1 and 2, provide complementary information on the state of the surface and are fused to derive internally consistent soil moisture estimates with quantified uncertainties. Field data from the TERENO test sites and MOSES initiative are used to validate the retrievals. In particular, cosmic-ray neutron sensing (CRNS) as a ground reference hold the benefit of involving spatial heterogeneity within soil moisture measurements. Additionally, both exponential filtering method and data assimilation schemes are applied to retrieve root zone soil moisture based on high-resolution surface soil moisture estimates. This project is embedded in the PhD College MoDEV.
Predicting vegetation transpiration from sap flow measurements using Machine Learning
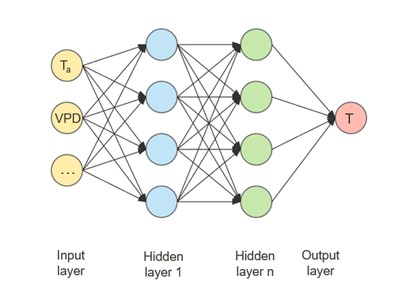
Evaporation from vegetation transpiration is a key process coupling the terrestrial energy, carbon and water cycles and plays an important role for several land-atmosphere feedbacks. While taking up the dominant proportion of total land evaporation, knowledge about global transpiration fluxes is still limited. Due to the inability to measure evaporation directly from space using remote sensing methods, we mostly rely on models and empirical partitioning methods to constrain transpiration. These estimates incorporate large uncertainty and differ greatly among each other. With the recently published SAPFLUXNET, a global compilation of whole-plant transpiration from sap flow measurements has been made available to the scientific community, providing the opportunity to improve our understanding of transpiration. By using state-of-the-art machine learning algorithms, we aim to bridge in-situ observations of sap flow with large-scale environmental drivers for transpiration from different sources such as ERA5-LAND and MODIS satellite observations. By employing an artificial neural network (ANN) we want to make predictions of transpiration on the large scale. This project is embedded in the PhD College MoDEV.
Quantifying the impact of climate change on crop yields
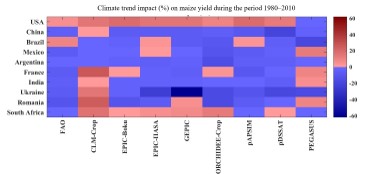
Climate change plays an important role in crop growth and crop yield, especially under global warming. It is necessary to quantify the climate change impacts on crop yield for agriculture improvement, and even maintaining food security in the world. We aim to quantify the climate change impact on crop yields, and make a reliable prediction of crop yield at global and regional scales. The main tools are machine learning methods and process-based crop models, based on which remote sensing data are combined to improve the model accuracy.
Contact: jian.peng@ufz.de