Microbial Data Science
Vision
The Microbial Data Science team cultivates an interdisciplinary systems biology approach wherein Microbial Ecology, Microbial Physiology, Bioinformatics, Artificial Intelligence, and Engineering converge. This convergence fosters innovative thinking aimed at developing “microbial systems digital twins” within both engineering and natural environments. Microbial systems digital tweens refer to virtual representations or simulations of microbial communities and their interactions within a given environment.
Mission
Microbial systems' digital twins are virtual representations or simulations of microbial communities and their interactions within specific environments. Our mission within the Microbial Data Science team at the UFZ is to advance the development of these digital twins. By doing so, we aim to replicate the behaviour and dynamics of authentic microbial ecosystems in silico. These digital twins will empower researchers to comprehend and explore the functional capabilities, responses to stimuli, and disruptions within microbial systems, without the necessity for extensive and costly experimental setups. Essentially, digital twins will facilitate scientists in exploring and manipulating microbial systems virtually, thus furnishing valuable insights across a spectrum of disciplines including environmental science, biotechnology, and medicine.
The Microbial Data Science team will embark on the generation of microbial systems' digital twins by delving into various data sources, encompassing (meta)genomics, (meta)transcriptomics, (meta)proteomics, metabolomics, environmental metadata, and text mining. Leveraging these diverse data sources, we will formulate concepts and theories to broaden the modelling of microbial communities, with a specific emphasis on capturing the vast diversity inherent in natural ecosystems by combining bioinformatics and artificial intelligence. Our focus will be on predicting and regulating ecosystem processes, particularly during disturbance events.
Research Topics
Our group strives to assess environmental health of terrestrials and man made environments by predicting how resilient/stable a microbial community is to specific disturbances. A special emphasis is put on the development of concepts and theories to scale microbial interactions to the real diversity found in nature.
The key research topics of the Microbial Data Science group are based on genetic potential of microbial communities, integration of multi-omics analysis and predictive biology. Currently these topics cover:
We are shedding light into the factors influencing the recovery of genomes from metagenomes towards the development of neutral and niche theories to study microbial interactions using multi-omics data.
• Expanding limits of detection of traditional Omics techniques: In collaboration with the Flow Cytometry group in our department, we are using a combination of fluorescence-activated cell sorting and metagenomics sequence to increase the limits of detection of metagenome assembled genomes in microbial communities.Use of 'omics' databases to elucidate long-time assumptions in microbiology.
• Scaling modeling of microbial interactions to the diversity found in natural ecosystems: Definition of concepts and theories to use partial genome-scale metabolic modeling as a tool to explore microbial community interactions.
• Species in the era of metagenome assembled genomes: We are developing techniques to exploit the diversity of metagenome assembled genomes by resolving species (and potentially strain) variation in complex microbial communities.
• Exploration of regulatory network in complex microbial communities: We are developing deep learning approaches to map transcription factors (and potentially their binding sites) in complex microbial communities.
• High-throughput genomic analysis: with the exponential increase of genomic (and multi-omics) data available in public repositories, we are creating user-friendly pipelines that can be used by microbiologists and microbial ecologists to analyze hundreds or thousands of genomes simultaneously.
We are developing tools to adapt predictive analytics to relevant questions and hypothesis relevant to microbiologists, environmental microbiologists and microbial ecologists.
• Prediction of health and dysbiosis states in microbial communities: We are developing pipelines to detect health and dysbiosis from multi-omics bioindicators using machine learning.
• Prediction of ecosystem services based on omics data: We are developing pipeline mixing traditional omics and machine learning to determine ecosystem services in a multi-omics perspective. We are currently using benzoate degradation and carbon fixation pathways as a model pathways.
• Prediction of trends in microbiology: We are developing machine learning approaches to understand trends in microbiology research from 1990 to 2020 and to predict the future trends for this field in the next 7 years.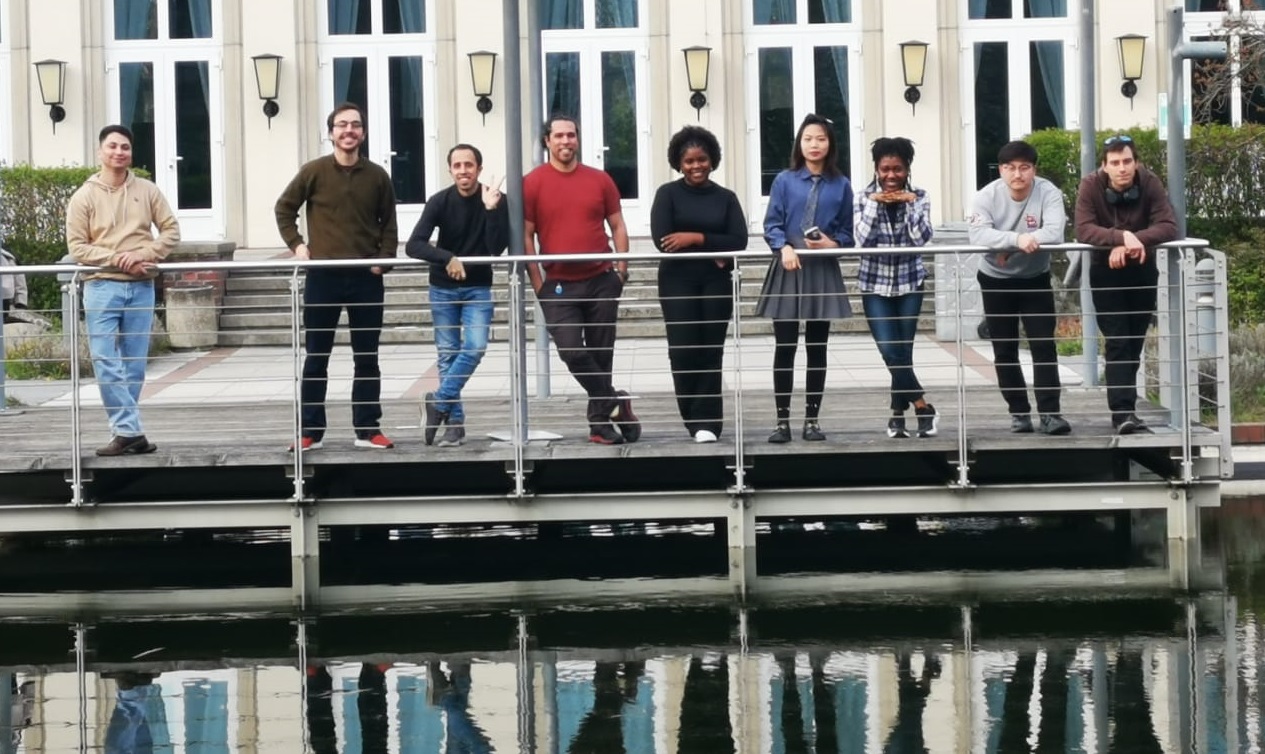
Postdoctoral / Research Associate
Mr. Jonas Coelho Kasmanas
Guest Scientists
Mrs. Dora Feng (until February 2025)
Mrs. Tianci Zhao (until July 2024)
Bachelor Student
Ana Julia Gonzalez Tendulini (until December 2024, USP, BR)
PhD Students
Anderson Paulo Avila Santos (Thesis defense, June 2024)
Faith Oni (Started October 2022)
Martin Bole (Started November 2022)
Sanchita Kamath (Started March 2023)
Asaad Ataa (Started October 2023)
Denis Tavares da Silva (Started February 2024)
- Interdisciplinary approach to understand microbial interactions in highly complex system.
- Genomics of microbial species using genomes metagenome-assembled genomes.
- Metabolic modeling of microbial systems where links between genes, reactions and metabolites are available.
- Development of concepts and theories to scale modeling of the molecular mechanisms of inter-species interactions to the diversity found in natural ecosystems.
- Open discussion with team members and collaborators to set goals and deadlines as well as to analyze progress of the work
- Each member of the team is responsible for a different set of skills and for connecting them to the different partners within team members of collaborators
- Out of the box thinking is stimulated
- Establishment of collaborations within UFZ, the Helmholtz family, German and international universities and research groups.
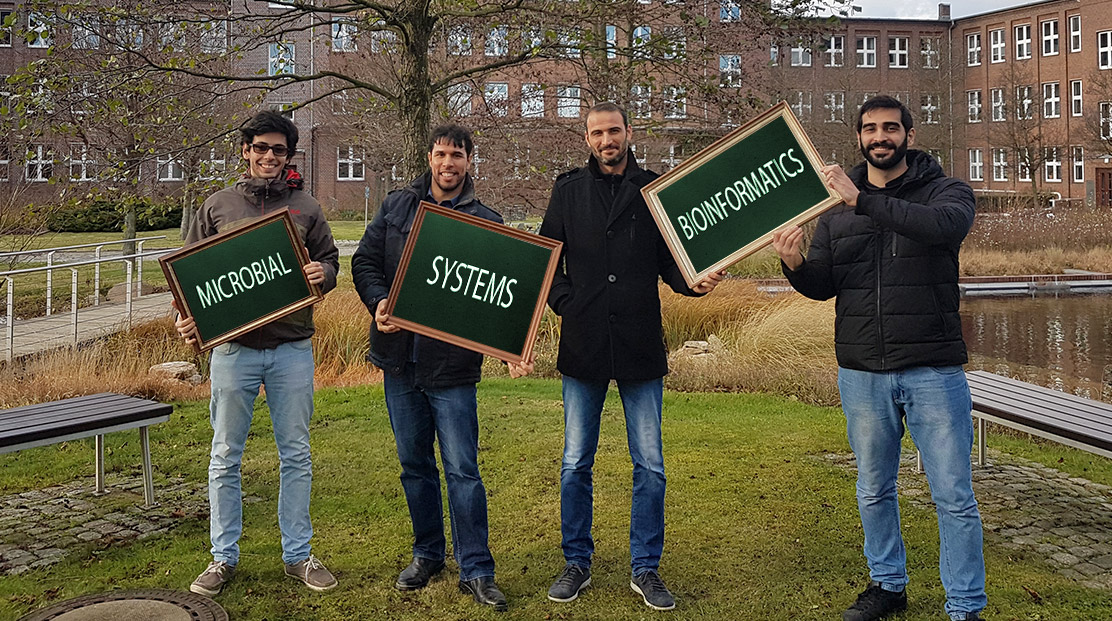