Multiscale Data Assimilation & Evaluation
- Multiscale and Multivariable Evaluation of Water Fluxes and States over European River Basins
- Multiscale and Multivariable Hydrologic Parameterization over Pan European River Basins
- Robust Framework for Parameter Inference over Large Domains
- Calibration of a hydrological model using patterns of satellite derived land surface temperature
- Data Assimilation and Model Calibration for Regional Soil Moisture
Multiscale and Multivariable Evaluation of Water Fluxes and States over European River Basins
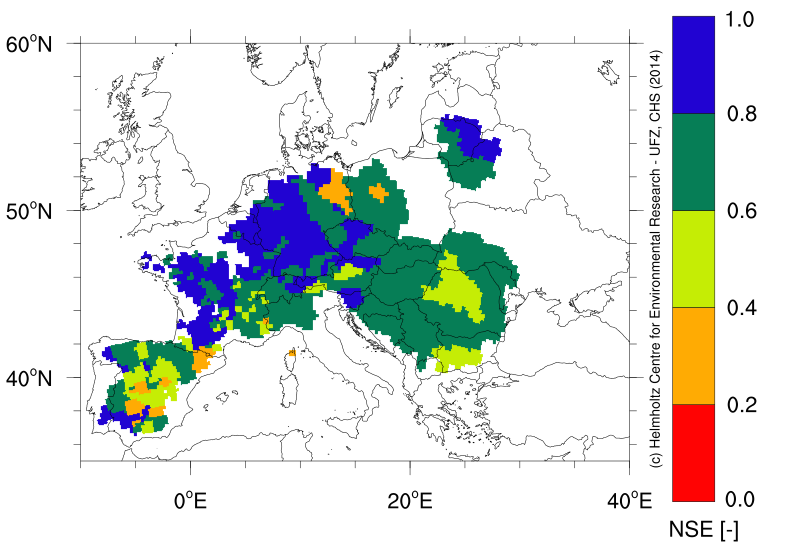
Developing the ability to predict streamflow at various scales is a key step for improving our understanding of the water balance and the skill of hydrologic models (HM). To cope with this grand challenge, we postulate that validating a HM only against an integral basin response such as streamflow is a necessary but not a sufficient condition to warranty the proper partitioning of incoming precipitation and net-radiation into different water storage components and fluxes.
Water fluxes and states variables are estimated for 350 basins within the Pan-EU domain with the mesoscale hydrologic model (mHM www.ufz.de/mhm). Its main features are the treatment of the sub-grid variability of input variables and model parameters and the possibility to estimate fluxes in nested-scales and/or in multiple basins keeping its regionalization coefficients unaltered across scales and basins. These essential features allow to simulate fluxes and states and to evaluate them with disparate sources of information such as satellite, streamflow, and eddy covariance data at their native resolutions. Therefore, we do not need ad-hoc rules to disaggregate/aggregate data for comparing model and observations at their native scales.
Multiscale and Multivariable Hydrologic Parameterization over Pan European River Basins
Besides traditional calibration of hydrologic model mHM against observed discharge, model parametrization is further constrained by a range of other variables available at different spatial scales: GRACE terrestrial water storage content (1o resolution), eddy flux data (500 m footprint) and ESA soil moisture product (0.25o resolution). Initial results shows that model parameterization constrained by streamflow only can not be outperformed. However, while parameterization based on complementary data sets leads to slight deterioration in streamflow performance, this marginal loss is balanced by improved simulation of other model states and fluxes. This becomes beneficial especially during the forecast applications, for which correct model initialization is crucial.
Robust Framework for Parameter Inference over Large Domains
Current computational limitations impose also seriously limitations to exhaustively investigate the parameter space of Land Surface models (LSM) over large domains (e.g. greater than half a million square kilometers). Addressing these challenges require holistic approaches that integrate the best techniques available for parameter estimation, sensitivity analysis, and the use of remotely sensed data at their native resolutions. An attempt to systematically address these issues is to combine sophisticated sampling techniques with the multiscale parameterisation technique (MPR) that links high resolution land surface characteristics with effective model parameters.
For more information, please contact: Rohini Kumar, Oldrich Rakovec, Luis Samaniego
Calibration of a hydrological model using patterns of satellite derived land surface temperature
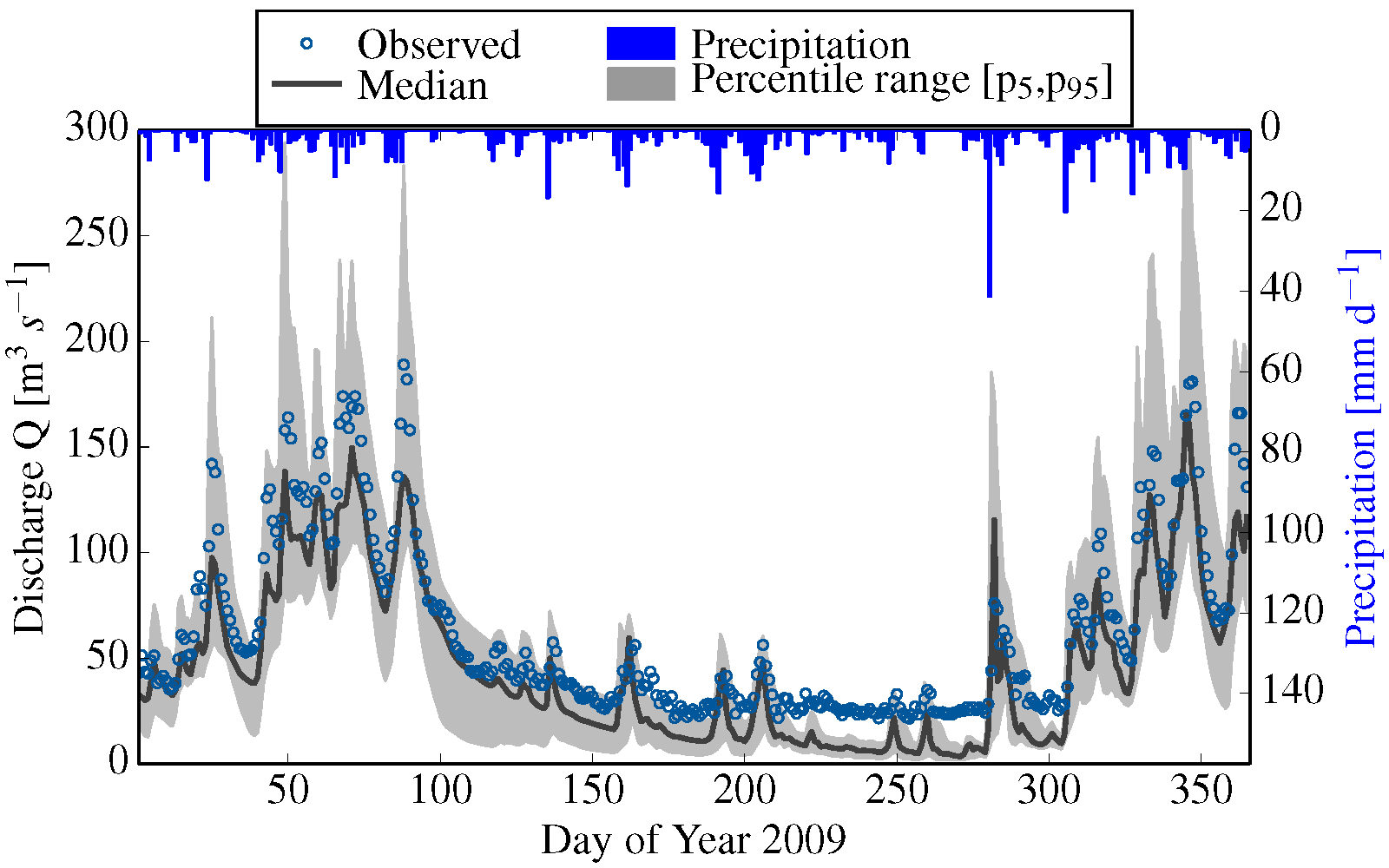
Hydrological models are usually calibrated against discharge and thus rely on gauging station information for estimating hydrological fluxes and state variables within the catchment of interest. But gauging data are rarely available and do only deliver information about spatially integrated signals of water fluxes within a catchment. In contrast, satellite data which include spatial distributed information are broadly available. Since satellite derived soil moisture and evapotranspiration (ET) data are prone to large uncertainty, more certain land surface temperature data are used in this study.
Estimated evapotranspiration of the catchment Neckar (size=12.700 km2) on July 1st, 2009 for the calibration against discharge (Q) and the calibration against discharge and landsurface termperature (Q & Ts) combined.
The objective of this study is to assess the predictive skill of satellite derived land surface temperature Ts regarding discharge by calibrating the distributed hydrological model mHM using Ts. The calibration of six catchments within Germany against Ts leads to Nash-Sutcliffe efficiencies (NSEs) of the estimated discharge between 0.3 and 0.6. While high flows are matched satisfactorily, the prediction of low flows is weak. Furthermore, a change of the spatial distribution of ET within the catchments is observed compared to calibrations against discharge only. The spatial variability of ET decreases compared to results of optimization against discharge. Further, model parameters related to ET are better constrained if considering both, land surface temperature and discharge, for the calibration of the model.
For more information, please contact: Matthias Zink
Data Assimilation and Model Calibration for Regional Soil Moisture
Cosmic-Ray Neutron Sensing with the TERENO:Rover
Measuring soil moisture with Cosmic Ray Sensors (CRS) is an emerging field of research, because the mesoscale footprint (~0.3 km²) is of great interest for land surface models that usually come with resolutions in between point measurements (e.g. TDR) and remote-sensing data.
We use the mesoscale Hydrological Model mHM to adapt the model parameters to the assimilated CRS data in order to (1) reduce the uncertainty of soil moisture predictions and (2) regionalise parameters for the conversion function from neutrons to soil moisture.
For further information please contact Martin Schrön