Essay
Environmental Perspectives 08/2018
How (un)certain is the future? Smart models in environmental research
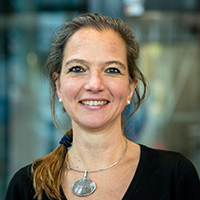
Prof. Dr. Sabine Attinger
Head of the Research Unit Smart Models / Monitoring and the Department Computational Hydrosystems
The theoretical physicist heads the UFZ "Smart Models and Monitoring" Research Unit and the Department of "Computational Hydrosystems". She is also the Chair of "Mathematical Modelling" in the Institute for Environmental Science and Geography at the University of Potsdam.
Prof. Dr. Sabine Attinger also heads and supports several scientific projects of the DFG and the BMBF. Since 2014, she has been speaker for the topic "From Observations to Predictions" in the Helmholtz "Terrestrial Environment" research programme.
Her current research focuses on spatially distributed predictive multiscale environmental models, their parametrisation and evaluation of the effects of uncertainties on model predictions. She collaborates with other scientists to predict future effects of hydrometeorological extremes on sectors of socioeconomic relevance (water supply, agriculture, urban areas).
She coordinates the activities of the UFZ related to monitoring and observing terrestrial systems either over the long term (eLTER, TERENO) or over the event-based timeline (MOSES). She is a member of the steering committee for the "Centre for Advanced System Understanding CASUS", a centre for digital interdisciplinary systems research in Görlitz, Germany. CASUS combines methods from mathematics, systems theory, data science and scientific computing in a single location to facilitate interdisciplinary rethinking of data-intensive systems research.
She is also actively involved in the "Helmholtz Information and Data Science Incubator" and the Helmholtz project "Digitalisation in Science Strategy". The application and development of data science methods and strategies of research data management are also topics in her everyday scientific life. She is also a member of the Helmholtz Working Group for Scientific Digitalisation in the Helmholtz Association, which directs the current and future activities of eight Helmholtz Centres in the context of the newly developed Helmholtz Strategy for Scientific Digitalisation.
Since the start of the 20th century, not only technology and the natural sciences have developed rapidly – mathematical models have also taken on increasing importance. The film "Hidden Figures" provides a strong image of how, in 1964, Katherine Johnson and the Rocket Girls sit by the dozens for days working with slide rules in open-plan offices to calculate orbits for the first NASA satellites. Today, we could do this with a smartphone in less than a second, but the mathematics behind it would be the same. It is computing power that has increased by a factor of 1010 over the past 50 years. This enables us to describe increasingly complex phenomena in computational models and to solve systems of equations increasingly quickly.
Environmental models deal with describing and predicting our environment, which is both a complex and sensitive system.
This step is associated with significant uncertainties, which is reflected in the result of the modelling – the prediction. Therefore, the more parameters the model includes, the more important it is to account for all combinations of parametric uncertainties in an overall uncertainty analysis. For example, in a weather forecast, we are already used to hearing not only a prediction of an amount of precipitation, but also the probability of its occurrence. This probabilistic approach, i.e. accounting for probability, is also widely used in infrastructure planning: For example, the risk of failure of the infrastructure can be accounted for when planning the overflow of dams. The 100-year probability of a flood height does not mean that it only occurs once every 100 years, but rather that there is a one percent probability that it will be exceeded in any year.
Environmental models deal with describing and predicting our environment, which is both a complex and sensitive system. Over the past 50 years, a well-founded set of modelling tools has been established and much experience has been gathered in their implementation in many areas of environmental research. In addition to mathematical equations that describe the system as well as possible, each model also requires initial values, boundary conditions and the model parameters already mentioned. It is still a big challenge to select these.
We at the UFZ have therefore been working specifically on model parametrisation over the past ten years. We have managed to achieve a new level of quality in hydrological modeling with the MPR approach (Multiscale Parameter Regionalisation). The concept here is to use relatively simple mathematical functions to link properties of areas such as topographic height, soil properties or vegetation, which satellites can measure at very high resolution, to the complex model parameter fields for which the details are unknown. The benefit is that instead of having to estimate several million unknown parameters as in conventional high-resolution models, only a few degrees of freedom of these functions need be estimated. This represents a true quantum leap in predictive ability. Furthermore, we use methods from theoretical physics to make our models scalable. In this way, the structure of the model parameter fields remains independent of the spatial resolution of the model, which is a further benefit.
We have managed to achieve a new level of quality in hydrological modeling with the MPR approach (Multiscale Parameter Regionalisation).
For example, this "smart" modelling approach enabled us to simultaneously calculate rainfall runoff and soil moisture for hundreds of European streams within all watershed areas for a period of 50 to 100 years, while simultaneously quantifying the uncertainty of the projections. The results are the focus of the following feature article. This article explains how different degrees of global warming can affect drought, low water levels and floods in Europe.
And what comes next? Given the projections, we will attempt to estimate the total economic costs of the projected impacts. But above all, we will help to develop mitigation or adaptation strategies, as the impacts of these projections would be considerable, especially in Central Europe: The consequences would be low water levels in the rivers, significantly restricted navigability and insufficient cooling capacity for power plants. At the same time, electric power consumption would increase drastically in the affected summer months, as the use of air conditioning in private homes would become the standard with daytime temperatures of up to 40 degrees. Agriculture would have to be converted to artificial irrigation in large parts of the Federal Republic of Germany, where irrigation from aquifers will not be possible everywhere for several months.
Science has the task of estimating these consequences as accurately as possible. For us model developers, this means that we will constantly improve our models, as accurate conclusions regarding the consequences are important. Ultramodern Earth observation systems will also play a significant part. The new era of data science will help in processing and analysing the resulting big data in order to efficiently incorporate it into our models.Cover story Umweltperspektiven 08/2018 (in German)
How (un)certain is the future? Smart models in environmental research