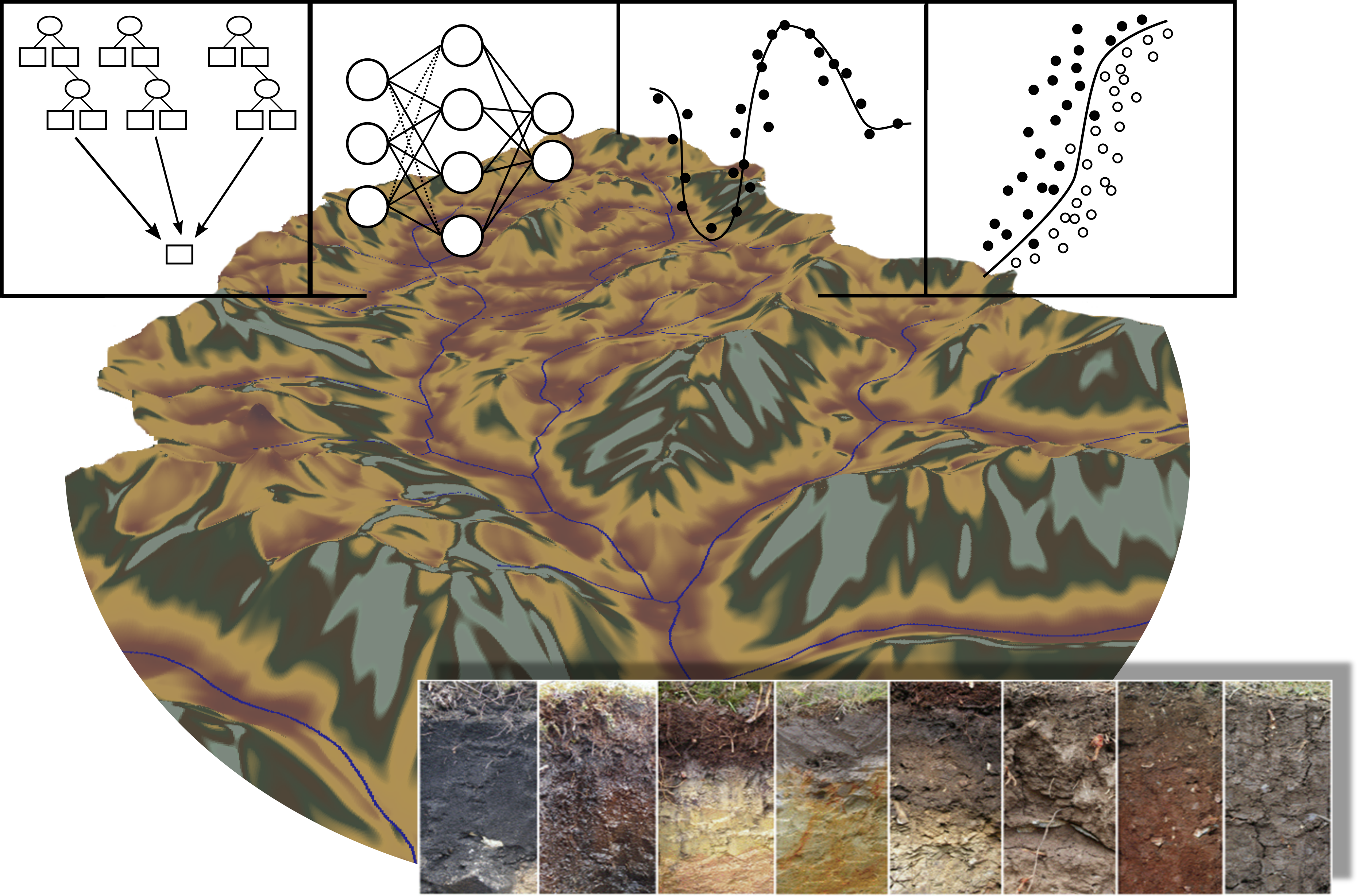
Pedometrics
At the UFZ working group Pedometrics it is our ambition to understand and model spatial soil distribution patterns and their interaction with the biosphere and hydrosphere.
Pedometrics is an interdisciplinary science integrating soil science, applied mathematics/ statistics and geoinformatics. The object of investigation is the spatial-temporal pedodiversity at multiple scales. Modelling approaches are used along with multiple aspects of soil sensing and geodata analysis. Common to all research approaches is that the quantification of the prediction uncertainty occupies an important space. Currently, the use of algorithms and optimization methods from the field of spatial Data Science is becoming increasingly important. The latter evolved from pattern recognition and computational learning theory and studies the application and construction of mathematical algorithms that can derive knowledge from complex data structures.
Scientists
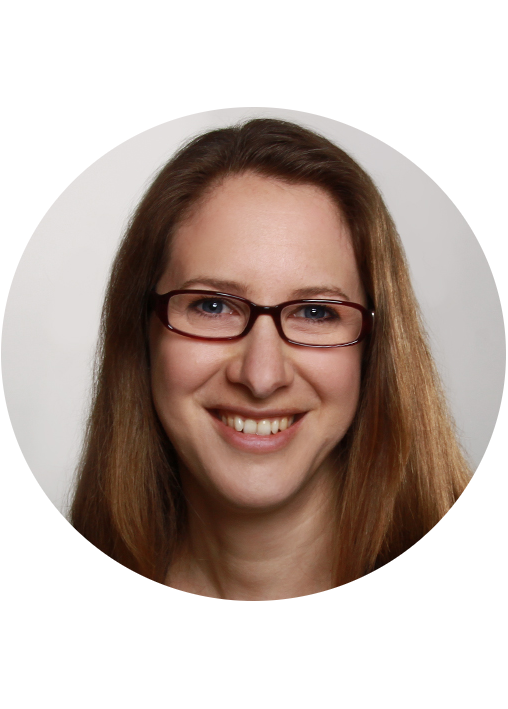
Dr. Mareike Ließ
Working group leader
Dr. Javier Reyes
PostDoc
Alumni
Ali Sakhaee
Anika Gebauer
Dr. Matteo Poggio
Carlos M. Guio Blanco
Monja Ellinger
Istvan Dunkl
Lisa Krieg
Tobias Koch
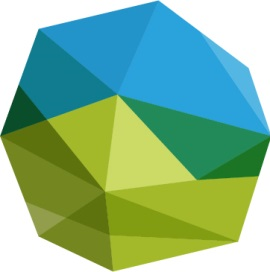
BONARES
Soil as a sustainable resource for the bioeconomy
Funding: Bundesministerium für Bildung und Forschung - BMBF
SoilSpace3D-DE
Creation of nationwide, spatially high-resolution soil information (2D, 3D) using various modelling approaches.
In cooperation with Thünen-Institut for Agricultural Climate Protection
SOCmonit
Monitoring of soil organic carbon using remote sensing and proximal soil sensing methods
Funding: Bundesministerium für Ernährung und Landwirtschaft - BMEL
2024 (5)
- Ließ, M. (2024):
Soil texture spatial rasters | Agricultural Soil-landscape of Germany
BonaRes 10.20387/bonares-ss02-6dys - Ließ, M., Sakhaee, A. (2024):
Deep learning with a multi-task convolutional neural network to generate a national-scale 3D soil data product: The particle size distribution of the German agricultural soil landscape
Agriculture-Basel 14 (8), art. 1230 10.3390/agriculture14081230 - Reyes, J., Ließ, M. (2024):
Spectral data processing for field-scale soil organic carbon monitoring
Sensors 24 (3), art. 849 10.3390/s24030849 - Reyes, J., Wiedemann, W., Brand, A., Franke, J., Ließ, M. (2024):
Predictive monitoring of soil organic carbon using multispectral UAV imagery: a case study on a long-term experimental field
Spat. Inf. Res. 32 , 683 - 696 10.1007/s41324-024-00589-7 - Sakhaee, A., Scholten, T., Taghizadeh-Mehrjardi, R., Ließ, M., Don, A. (2024):
Spatial prediction of organic matter quality in German agricultural topsoils
Agriculture-Basel 14 (8), art. 1298 10.3390/agriculture14081298
2023 (1)
- Reyes, J., Ließ, M. (2023):
On-the-go Vis-NIR spectroscopy for field-scale spatial-temporal monitoring of soil organic carbon
Agriculture-Basel 13 (8), art. 1611 10.3390/agriculture13081611
2022 (5)
- Dunkl, I., Ließ, M. (2022):
On the benefits of clustering approaches in digital soil mapping: an application example concerning soil texture regionalization
Soil 8 (2), 541 - 558 10.5194/soil-8-541-2022 - Gebauer, A., Sakhaee, A., Don, A., Poggio, M., Ließ, M. (2022):
Topsoil texture regionalization for agricultural soils in Germany – an iterative approach to advance model interpretation
Front. Soil Sci. 1 , art. 770326 10.3389/fsoil.2021.770326 - Ließ, M. (2022):
3D soil parameter space of the agricultural landscape [Germany, Version 2]
BonaRes 10.20387/bonares-13qm-mw25 - Ließ, M. (2022):
Modeling the agricultural soil landscape of Germany—A data science approach involving spatially allocated functional soil process units
Agriculture-Basel 12 (11), art. 1784 10.3390/agriculture12111784 - Sakhaee, A., Gebauer, A., Ließ, M., Don, A. (2022):
Spatial prediction of organic carbon in German agricultural topsoil using machine learning algorithms
Soil 8 (2), 587 - 604 10.5194/soil-8-587-2022
2021 (2)
- Bartkowski, B., Droste, N., Ließ, M., Sidemo-Holm, W., Weller, U., Brady, M.V. (2021):
Payments by modelled results: A novel design for agri-environmental schemes
Land Use Pol. 102 , art. 105230 10.1016/j.landusepol.2020.105230 - Ließ, M., Gebauer, A., Don, A. (2021):
Machine learning with GA optimization to model the agricultural soil-landscape of Germany: An approach involving soil functional types with their multivariate parameter distributions along the depth profile
Front. Environ. Sci. 9 , art. 692959 10.3389/fenvs.2021.692959
2020 (3)
- Gebauer, A., Ellinger, M., Brito Gomez, V.M., Ließ, M. (2020):
Development of pedotransfer functions for water retention in tropical mountain soil landscapes: spotlight on parameter tuning in machine learning
Soil 6 (1), 215 - 229 10.5194/soil-6-215-2020 - Ließ, M. (2020):
At the interface between domain knowledge and statistical sampling theory: Conditional distribution based sampling for environmental survey (CODIBAS)
Catena 187 , art. 104423 10.1016/j.catena.2019.104423 - Zhang, X., Wendroth, O., Matocha, C., Zhu, J., Reyes, J. (2020):
Assessing field-scale variability of soil hydraulic conductivity at and near saturation
Catena 187 , art. 104335 10.1016/j.catena.2019.104335
2019 (6)
- Bartkowski, B., Droste, N., Ließ, M., Sidemo-Holm, W., Weller, U., Brady, M.V. (2019):
Implementing result-based agri-environmental payments by means of modelling
UFZ Discussion Papers 5/2019
Helmholtz-Zentrum für Umweltforschung - UFZ, Leipzig, 28 pp. - Ellinger, M., Merbach, I., Werban, U., Ließ, M. (2019):
Error propagation in spectrometric functions of soil organic carbon
Soil 5 (2), 275 - 288 10.5194/soil-5-275-2019 - Gebauer, A., Brito Gómez, V.M., Ließ, M. (2019):
Optimisation in machine learning: An application to topsoil organic stocks prediction in a dry forest ecosystem
Geoderma 354 , art. 113846 10.1016/j.geoderma.2019.07.004 - Reyes, J., Wendroth, O., Matocha, C., Zhu, J. (2019):
Delineating site-specific management zones and evaluating soil water temporal dynamics in a farmer’s field in Kentucky
Vadose Zone J. 18 (1), art. 180143 10.2136/vzj2018.07.0143 - Vogel, H.-J., Eberhardt, E., Franko, U., Lang, B., Ließ, M., Weller, U., Wiesmeier, M., Wollschläger, U. (2019):
Quantitative evaluation of soil functions: potential and state
Front. Environ. Sci. 7 , art. 164 10.3389/fenvs.2019.00164 - Wiesmeier, M., Urbanski, L., Hobley, E., Lang, B., von Lützow, M., Marin-Spiotta, E., van Wesemael, B., Rabot, E., Ließ, M., Garcia-Franco, N., Wollschläger, U., Vogel, H.-J., Kögel-Knabner, I. (2019):
Soil organic carbon storage as a key function of soils - A review of drivers and indicators at various scales
Geoderma 333 , 149 - 162 10.1016/j.geoderma.2018.07.026
2018 (1)
- Guio Blanco, C.M., Brito Gomez, V.M., Crespo, P., Ließ, M. (2018):
Spatial prediction of soil water retention in a Páramo landscape: methodological insight into machine learning using random forest
Geoderma 316 , 100 - 114 10.1016/j.geoderma.2017.12.002
2017 (2)
- Jeong, G., Choi, K., Spohn, M., Park, S.J., Huwe, B., Ließ, M. (2017):
Environmental drivers of spatial patterns of topsoil nitrogen and phosphorus under monsoon conditions in a complex terrain of South Korea
PLOS One 12 (8), e0183205 10.1371/journal.pone.0183205 - Jeong, G., Oeverdieck, H., Park, S.J., Huwe, B., Ließ, M. (2017):
Spatial soil nutrients prediction using three supervised learning methods for assessment of land potentials in complex terrain
Catena 154 , 73 - 84 10.1016/j.catena.2017.02.006
2016 (1)
- Ließ, M., Schmidt, J., Glaser, B. (2016):
Improving the spatial prediction of soil organic carbon stocks in a complex tropical mountain landscape by methodological specifications in machine learning approaches
PLOS One 11 (4), e0153673 10.1371/journal.pone.0153673