Pedometrics
At the UFZ working group Pedometrics it is our ambition to understand and model spatial soil distribution patterns and their interaction with the biosphere and hydrosphere.
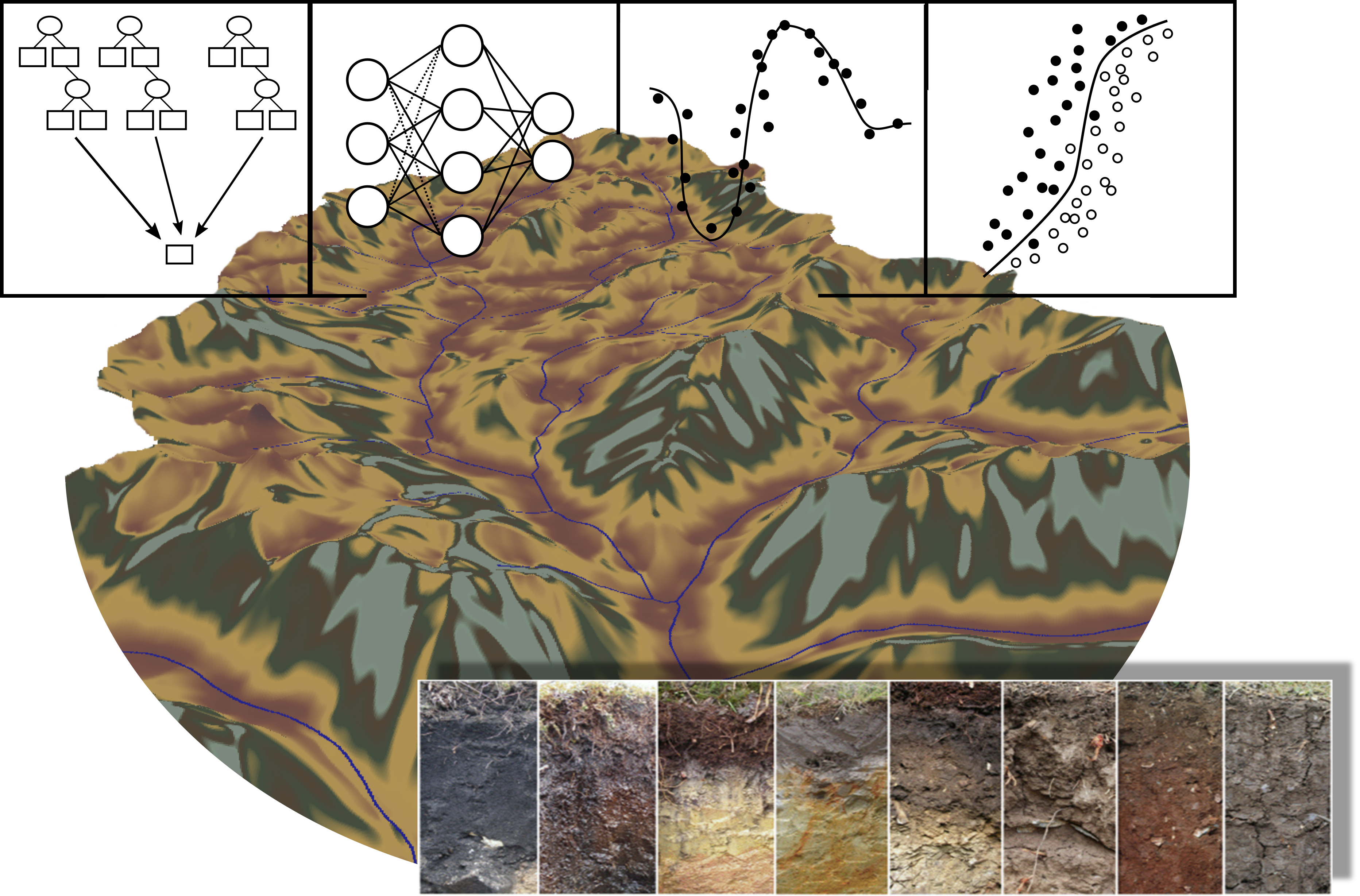
Head
Dr. Mareike Ließ
Scientists
Anika Gebauer
Ali Sakhaee (external)
Dr. Javier Reyes
Dr. Matteo Poggio
Student Research
Lisa Krieg
Tobias Koch
Pedometrics is an interdisciplinary science integrating soil science, applied mathematics/ statistics and geoinformatics. The object of investigation is the spatial-temporal pedodiversity at multiple scales. Modelling approaches are used along with multiple aspects of soil sensing and geodata analysis. Common to all research approaches is that the quantification of the prediction uncertainty occupies an important space. Currently, the use of algorithms and optimization methods from the field of spatial Data Science is becoming increasingly important. The latter evolved from pattern recognition and computational learning theory and studies the application and construction of mathematical algorithms that can derive knowledge from complex data structures.
Current projects
SoilSpace3D-DE
Research cooperation with the Thünen Institute of Climate-Smart Agricultural to create nationwide, spatial high-resolution soil information (2D, 3D) using different modeling approaches. Process modeling approaches of the agricultural landscape require high-resolution soil information of the multivariate soil parameter space. In order to generate these parameter fields, it is necessary to understand spatial soil distribution patterns and to model them using machine and deep learning algorithms.
SOCmonit
Under a detrimental agricultural soil management, soil organic carbon (SOC) is faster released into the atmosphere than is required for its sequestration. Accordingly, the long-term maintenance of SOC promoting management is essential. In the SOCmonit project, soil spectroscopy is used to develop a spatio-temporal monitoring approach. The sensors and platforms for remote and proximal sensing used in the project generate diverse data formats with various information contents with regards to the target variable. The data processing steps as well as the steps involved in modeling for the SOC prediction are implemented in open source software, automated and documented in a comprehensible manner. The project is carried out in cooperation with the company RSS - Remote Sensing Solutions GmbH. SOCmonit
BonaRes
Soil as a sustainable resource for the bioeconomy. Soil fertility forms the basis for sustainable plant production. Soils not only have to guarantee marketable yields, they also have to provide diverse ecosystem services. 10 interdisciplinary collaborative projects and the BonaRes Centre conduct research under the umbrella of BonaRes. The BonaRes Centre deals with the transfer of existing and newly generated knowledge about soil functions into scientifically based decision support tools for soil management. The main tasks are to establish the necessary data infrastructures, model concepts and assessment instruments for sustainable soil management. https://www.bonares.de/
HI-CAM, Project 7.1 - Agricultural and aquatic systems
The Helmholtz Climate Initiative develops climate mitigation and adaptation strategies. Research expertise is bundled in interdisciplinary cooperation between a wide variety of Helmholtz centers and external partners in order to develop recommendations for decision-makers in politics and society in the context of climate change. In project 7.1 the selected modeling approach is used to link soil process understanding to national soil databases in order to create spatially continuous, high-resolution soil information. The focus is on soil properties, which are considered suitable indicators for agricultural productivity - in particular soil water storage. The project contributes to the development of sustainable adaptation strategies for agriculture in response to climate change. This is done at national scale (Germany), taking into account regional features with regards to soil distribution. HI-CAM
Recent puplications
Gebauer, A., Sakhaee, A., Don, A., Poggio, M., Ließ, M., 2022. Topsoil Texture Regionalization for Agricultural Soils in Germany — An Iterative Approach to Advance Model Interpretation. Front. Soil Sci. 1:770326. https://doi.org/10.3389/fsoil.2021.770326
Ließ, M., Gebauer, A., Don, A., 2021. Machine learning with GA optimization to model the agricultural soil-landscape of Germany: An approach involving soil functional types with their multivariate parameter distributions along the depth profile. Front. Environ. Sci. 9:692959. https://doi.org/10.3389/fenvs.2021.692959
Bartkowski, B., Droste, N., Ließ, M., Sidemo-Holm, W., Weller, U., Brady, M.V., 2021. Payments by modelled results: A novel design for agri-environmental schemes. Land Use Policy, 102, 105230. https://doi.org/10.1016/j.landusepol.2020.105230
Gebauer, A., Ellinger, M., Brito Gómez, V.M., Ließ, M., 2020. Development of pedotransfer functions for water retention in tropical mountain soil landscapes: spotlight on parameter tuning in machine learning. Soil, 6, 215–229. https://doi.org/10.5194/soil-6-215-2020
Ließ, M., 2020. At the interface between domain knowledge and statistical sampling theory: Conditional distribution based sampling for environmental survey (CODIBAS). Catena, 187,104423. https://doi.org/10.1016/j.catena.2019.104423
Ellinger, M., Merbach, I., Werban, U., Ließ, M., 2019. Error propagation in spectrometric functions of soil organic carbon. Soil, 5, 275-288. https://doi.org/10.5194/soil-5-275-2019
Gebauer, A., Brito Gómez, V.M., Ließ, M., 2019. Optimisation in machine learning: An application to topsoil organic carbon stocks prediction in a dry forest ecosystem. Geoderma, 354, 113846. https://doi.org/10.1016/j.geoderma.2019.07.004
Stein,S., Eberhardt, E., Grosse, M., Helming, K., Hierold, W., Hoffmann, C., Kühnert, T., Ließ, M., Russel, D.J., Schulz, S., Specka, X., Svoboda, N., Zoarder, M.A.M., Heinrich, U., 2019. Report on available soil data for German agricultural areas, 2018. BonaRes Series 2019/01. BonaRes Centre for Soil Research. DOI: 10.20387/BonaRes-CD4Q-1PEM
Vogel H-J, Eberhardt E, Franko U, Lang B, Ließ M, Weller U, Wiesmeier M, Wollschläger U, 2019. Quantitative evaluation of soil functions: potential and state. Frontiers in Environmental Science, 7 (164), https://doi.org/10.3389/fenvs.2019.00164
Wiesmeier, M., Urbanski, L., Hobley, E., Lang, B., von Lützow, M., Marin-Spinotta, E., van Wesemael, B., Rabot, E., Ließ, M., Garcia-Franko, N., Wollschläger, U., Vogerl, H.-J., Kögel-Knabner, I., 2019. Soil organic carbon storage as a key function of soils - A review of drivers and indicators at various scales. Geoderma, 333, 149-162. https://doi.org/10.1016/j.geoderma.2018.07.026