Vegetation
Remote sensing of vegetation at UFZ focusses on the interactions of land-use change, climate and vegetation on the basis of remotely sensed imagery. Here, the main interests are
- extracting phenological metrics from optical-reflective satellite data
- measure local forest structures
- deriving vegetation status from hyperspectral imagery
- bridging the gap between satellite and ground observations
- estimating plant productivity from hyperspectral data and
- setting-up a satellite product validation test sites
Remote Sensing data allow to measure local forest structures.
We use the forest modell to find connections between forest structure and the forest biomass, the degree of disturbance, as well as the productivity and the carbon cycle. The applied remote sensing data are either LiDAR data, multispectral data or aerial photographs. LiDAR data are obtained with an own specialized unmanned aerial vehicle - UAV (Quadrocopter Microdrones „md4-1000“). Additionally, the UAV can be equipped with a multispectral camera, a RGB camera and a thermal camera. LiDAR data are used to detect individual tree tops, tree species and gaps. This structural information can answer various ecological questions. Further application fields for the UAV can be agricultural sites, savannas or anthropogenically influenced edge areas of forests.
Extracting & simulating phenological metrics
The focus within vegetation phenology is on analysing the response of spring time phenology to climate change (Badeck et al. (2004)) using ground and satellite observations. We also assessed the influence of heterogeneous landscapes on computed green-up dates (Doktor et al. (2009)) and analysed trends of computed green-up dates on a European scale (Doktor et al. (2011)). A variety of methods to extract phenological metrics has been implemented in R package ’phenex’ to be of public use. Modelling of phenological phases of forest tree species (temperature and day-length driven, Schaber & Badeck (2003)) is done with the R-package ‘phenmod’. Here, the impact of several future climate scenarios on forest phenology has been analysed (Lange et al. (2016), under revision).
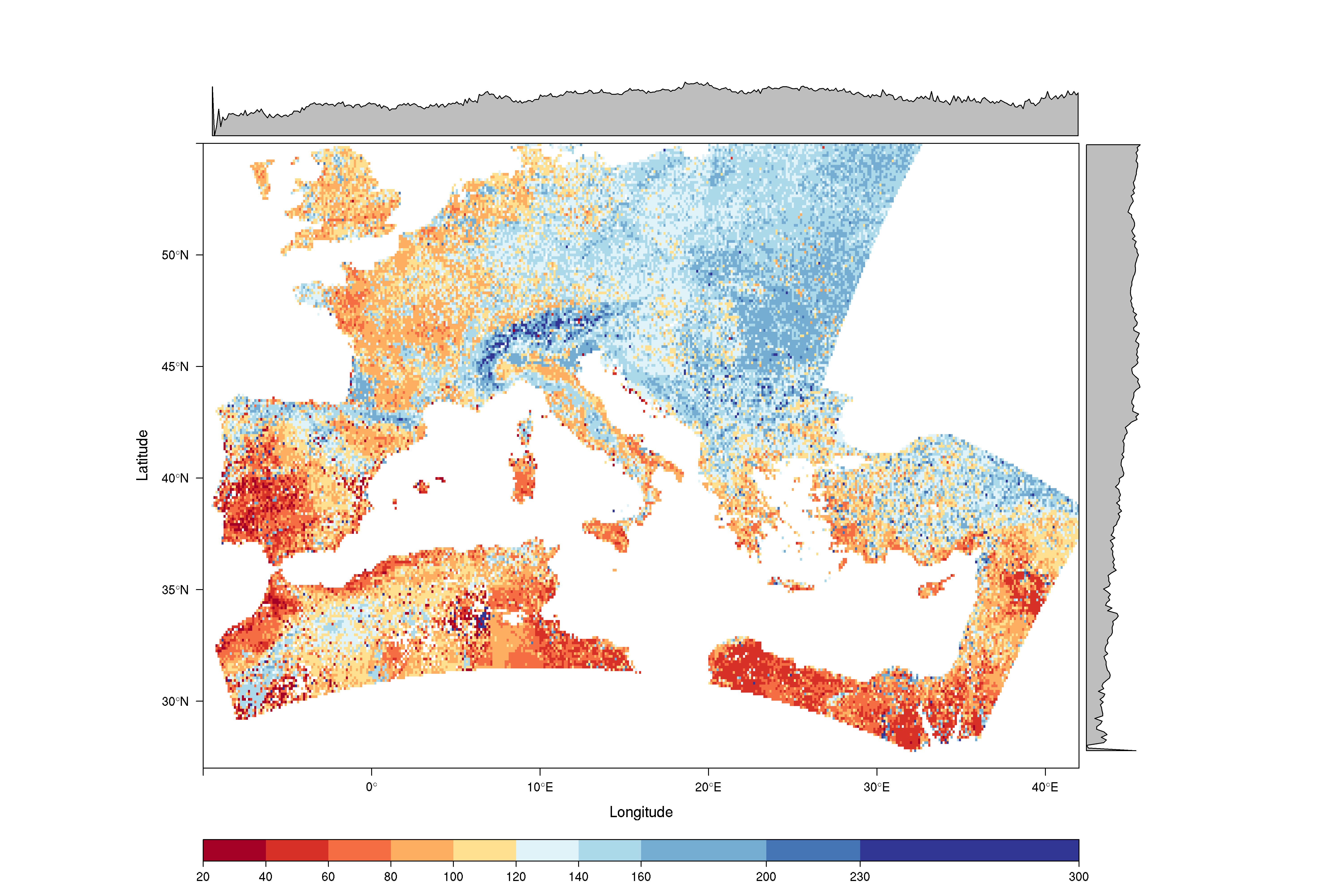
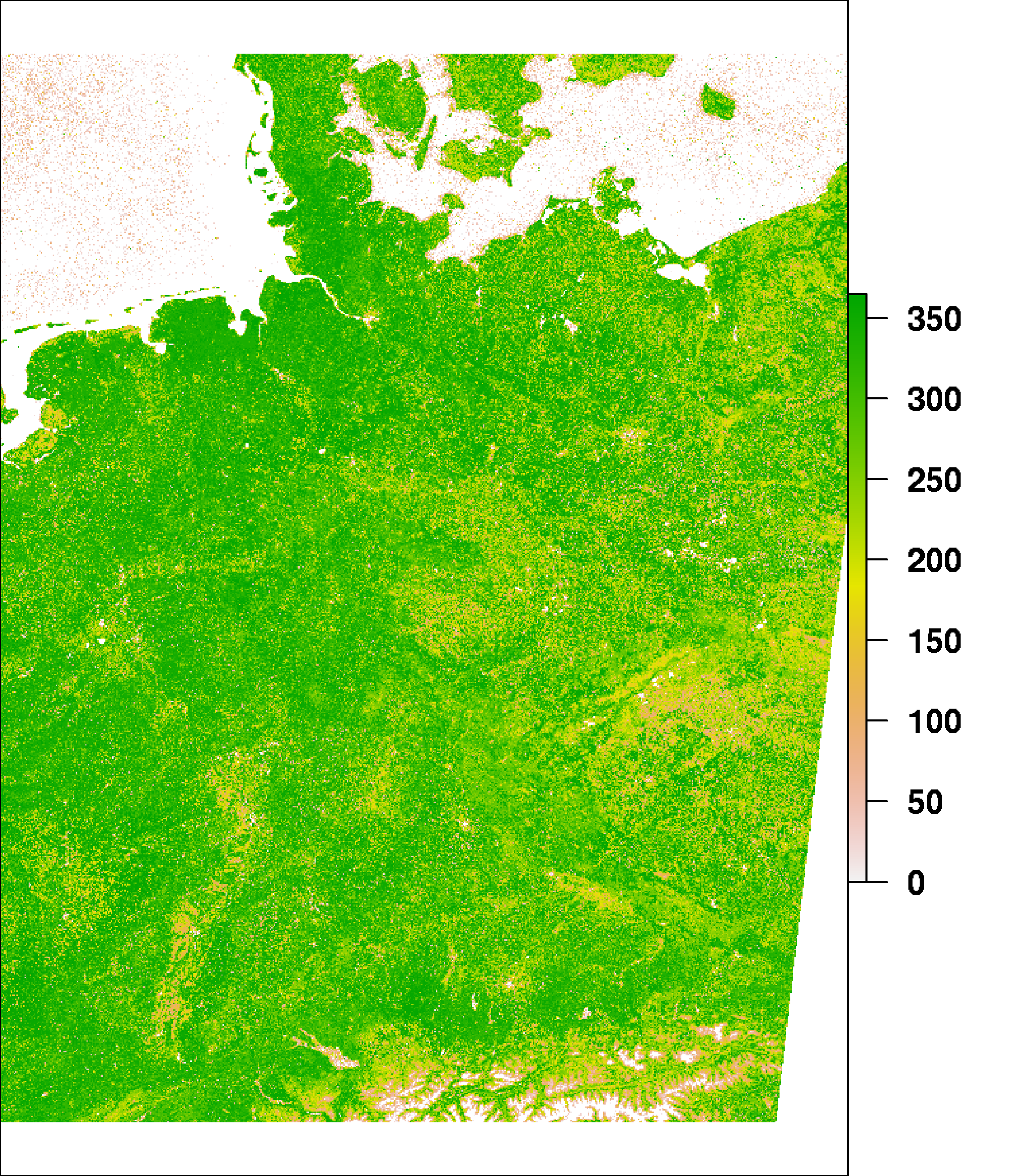
Extraction of biopyhsical vegetation variables
Here, UFZ relies on airborne and ground hyperspectral data acquisitions in order to:
1) Derive Essential Biodiversity and Essential Climate Variables (EBV & ECV) e.g. canopy chlorophyll content (Cab), Leaf Area Index (LAI); Doktor & Thurner (2014)
2) assess whether these EBV & ECV are captured differently by remotely sensed imagery at different spatial resolutions
3) map natural habitats / biodiversity: Luft et al., 2016; Neumann et al., 2015; Feilhauer et al. 2014
4) derive pollination types: Feilhauer, Skidmore, Schmidltein, Doktor (in revision)
5) classify species-rich broadleaf forests (Richter et al.; under review)
These sensors have also been used under controlled conditions in the laboratory (UFZ field research station Bad Lauchstädt, https://www.ufz.de/index.php?en=39922) to investigate the effect of water and drought stress scenarios on measured reflection profiles. Spectral measurements in the field and laboratory are accompanied by in-situ observations of e.g. Chlorophyll (Spad), LAI (LAI 2000), fluorescence/gas-exchange (Licor 6400) or soil moisture (TDR). As a result, UFZ acquires systematically scale-specific hyperspectral data (Lausch et. al, 2012/2013) to facilitate up-scaling.
A processing chain is in use for airborne raw hyperspectral data: radiometric correction, atmospheric correction, geometric correction, ortho-rectification relying on software from in-house developments, cooperations (GFZ) and ATCOR4 / Envi (e.g. Rogaß et al., 2011). Due to the multi-dimensionality and complexity of used datasets a range of (geo-) statistical and machine learning methods are routinely employed as well parallel processing on the UFZ cluster. When extracting biophysical plant variables radiative transfer models are indespensible. Here, our 'work-horses' are PROSAIL, SLC (Soil Leaf Canopy) and more recently DART (Discrete Anisotropic Radiative Transfer, http://www.cesbio.ups-tlse.fr/us/dart/dart_model.html). Radiative transfer model inversion are with PCA related methods, randomForest (Doktor & Thurner, 2014) and SVR (Preidl & Doktor, 2011).
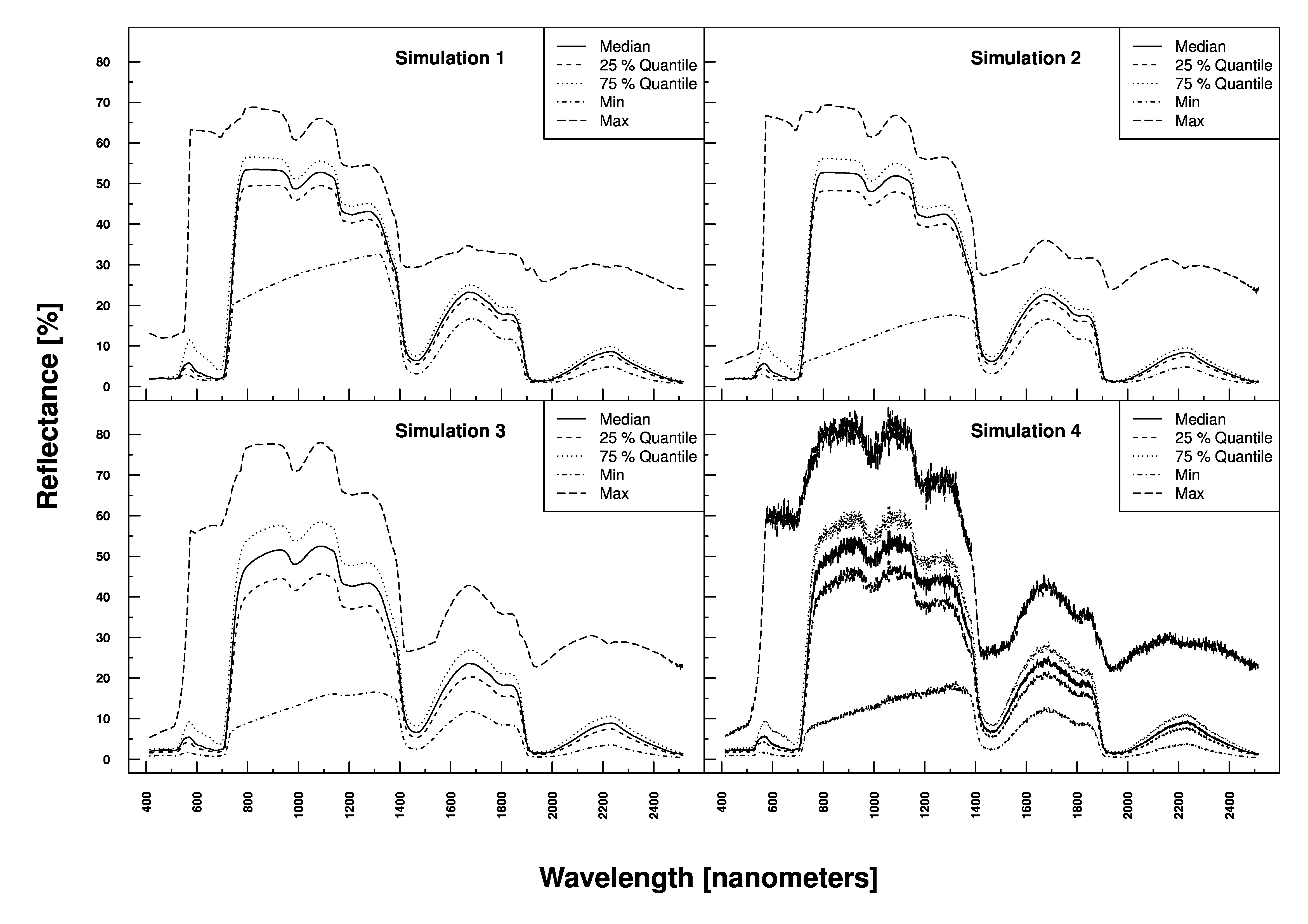
Satellite Sensors:
NOAA AVHRR (NOAA-11 - NOAA-18)
MODIS Terra & Aqua
Landsat TM, ETM, Landsat 8
RapidEye
(Airborne) imaging hyperspectral sensors
HySpex VNIR1800/SWIR384
AISA Eagle/Hawk
Spectrometers
ASD FieldSpec Pro 3/4
UAV Sensors:
LiDAR (SICK LD-MRS: Multi-layer, multi-echo laser scanner)
Multispectral Camera (Tetracam Mini MCA 6)
RGB Camera (Sony Alpha Nex7)
Thermal Radiometric Camera (Flir Tau2)
Sensor dependent:
- from local scale (30 * 30 m) to continental scale (e.g. Europe)
More specifically:
Tropical Forests - CTFS-Plots
Kilimanjaro - Tanzania
Loja - Ecuador
Hainich - UNESCO World Heritage Site - Germany
BIOTREE forest experiment - Germany
Traunstein - Germany (mixed forest)
Hohes Holz - Germany (deciduous forest + grassland)
LTER sites within Tereno 'Mitteldeutschland' - Germany (grassland, floodplains)
Harz mountains - Germany (agriculture, soil, forest)
Bad Lauchstädt - Germany (agriculture)
FORMIND
- forest mixture individual forest model
HGF-EDA - Helmholtz Alliance: Remote Sensing and Earth System Dynamics
'Ecopotential' (EU project within Horizon 2020), WP 4: 'Derivation of bio-physical variables from remotely sensed imagery'
Runtime: 2015-2019
Amount: ~130.000 € (1 Postdoc), Total project ~15 Mio €
'Feasibility study to derive nature protection criteria within the planning requirements of the federal network development'.
Runtime: 2015-2016
Amount: ~30.000 €
Funded by: Federal Agency for Nature Conservation (BfN)
'PhenoS - Optimisation of land-use classification based on Sentinel-2 phenological information of high temporal density'
Runtime: 2013-2016
Amount: ~ 143.000 €, 1 PhD
Funded by: BMWi (Federal Ministry of Economic Affairs and Energy) program 'Preparation of the scientific and economic exploitation of the Sentinel missions and national missions'
'Fusion of radar (L-band) and hyperspectral data to derive biomass, leaf area index and vegetation disturbance'. Within WP 'Biosphere'
Runtime: 2013-2016
Amount: ~ 120.000 €, 1 PhD
Funded by: Helmholtz-Allianz ‘Remote Sensing and Earth System Dynamics’
'Validation of Sentinel products on the basis of continuous spectral and micro-meteorological measurements'
Runtime: 2012-2015
Amount: ~ 170.000 €, 1 PhD
Funded by: BMWi (Federal Ministry of Economic Affairs and Energy) program 'Preparation of the scientific and economic exploitation of the Sentinel missions and national missions'
'Methods to derive functional dependencies of ecosystem processes within hyperspectral data of different spatial resolution'
Runtime: 2010-2013
Amount: ~ 130,000 €, 1 PhD
Funded by: BMWi (Federal Ministry of Economic Affairs and Energy) program 'Development of methods and algorithms for data analysis in preparation of the EnMAP mission'
ACROSS, Advanced Remote Sensing - Ground Truth Demo and Test Facilities (https://www.ufz.de/index.php?de=36778)
Funded by: Helmholtz Association
Research Group Kilimanjaro
STRUCTURE - The effects of land-use and structural heterogeneity on biodiversity: A new assessment method using cost-effective remote sensing and fine-scale pattern analysis (2008-2010).
Shugart, H.H., Asner, GP., Fischer, R., Huth, A., Knapp, N. Toan, T.L. & Shuman, J.K. (2015) Computer and remote-sensing infrastructure to enhance large-scale testing of individual-based forest models. Frontiers in Ecology and the Environment, 13, 503–511.
Getzin, S., Wiegand, K., Wiegand, T., Yizhaq, H., von Hardenberg, J. & Meron, E. (2015) Adopting a spatially explicit perspective to study the mysterious fairy circles of Namibia. Ecography, 38, 1-11.
Getzin, S., Nuske, R.S. & Wiegand, K. (2014) Using unmanned aerial vehicles (UAV) to quantify spatial gap patterns in forests. Remote Sensing, 6, 6988-7004.
Pütz, S., Groeneveld, J., Henle, K., Knogge, C., Martensen, A.C., Metz, M., Metzger, J.P., Ribeiro, M.C., de Paula, M.D., Huth, A. (2014) Long-term carbon loss in fragmented neotropical forests. Nature Communications, 5:5037.
Getzin, S., Wiegand, K. & Schoening, I. (2012) Assessing biodiversity in forests using very high-resolution images and unmanned aerial vehicles. Methods in Ecology and Evolution, 3, 397-404.
Köhler, P. & Huth, A. (2010) Towards ground-truthing of spaceborne estimates of above-ground life biomass and leaf area index in tropical rain forests. Biogeosciences, 7, 2531-2543
Getzin, S., Wiegand, K., Schumacher, J. & Gougeon, F.A. (2008) Scale-dependent competition at the stand level assessed from crown areas. Forest Ecology and Management, 255, 2478-2485.
Moustakas, A., Wiegand, K., Getzin, S. , Ward, D., Meyer, K.M., Guenther, M. & Mueller, K.-H. (2008) Spacing patterns of an Acacia tree in the Kalahari over a 61-year period: how clumped becomes regular and vice versa. Acta Oecologica, 33, 355-364.
- optical-reflective remote sensing (multi-spectral satellite & airborne hyperspectral)
- extraction of phenological metrics
- derivation of bio-physical plant parameters (EBV & ECV)
- phenological modelling
- data acquisition and data validation
- processing of airborne hyperspectral data
Forest modelling, analysis of satellite data and LiDAR data
Forest modelling, analysis of satellite data and LiDAR data
UAV-based spatial analysis of LiDAR, photo, thermal, and multispectral data